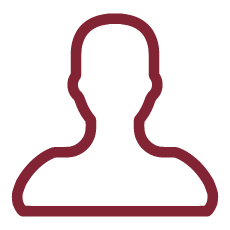
De-duplication (record linkage or entity resolution) is the process of merging together potentially noisy lists, data sets, or databases, often in the absence of a unique identifier, both to remove duplicated information and to increase the informative content of each single file. In fact, from a statistical perspective, performing de-duplication is paramount to obtaining a more reliable and informative reference data set. Indeed, on one hand, the identification of duplications of the same entity would allow to increase the quality of the information associated to it. On the other hand, merging different files, once the common entities have been correctly detected, leads to a new, larger and richer data set. This new data set may be suitable to perform accurate model-based statistical analyses via the additional information which could not be extracted from a single data set, because the original data may not comprise some of the model variables. When unique identifiers are known exactly, the linkage process can be accomplished without errors. However, in practice, unique identifiers are rarely available and the researcher must deal with the uncertainty related to the linking step. The problem of how to account for the matching uncertainty has then caused an active line of recent research among the statistical, the machine learning, and the computer science communities. In fact, in practical applications of record linkage procedures, the concrete possibility to make wrong matching decisions should be accounted for, especially when the result of the linking step, namely the fused data set, will be used for further statistical analyses, such as regression, capture-recapture methods or small area estimation. In this project we will develop a unified Bayesian framework for population size estimation and small area estimation by using multiple files that require a preliminary de-duplication process.
We believe that the introduction of Bayesian ideas in the practice of record linkage and inference with de-duplicated data sets will be highly beneficial and relevant for many reasons. In fact record linkage is not a free-error technique. Frequentist methods, that correct for the biases due to linkage errors when carrying out inferential analysis, assume that the matching and the inferential processes are two well separate and sequential steps. In our opinion such approach makes more difficult the subsequent model estimation which can only benefit from secondary correction techniques. On the other side, Bayesian models can safely take into account the decision uncertainty related to matching status of each record pair. By the joint modelling of the variables involved in the de-duplication process and the key variables, this uncertainty is naturally propagated in the statistical model estimation. In addition, we argue that this joint modelling approach, by exploiting the additional information provided by the inferential step also in the fitting of the record linkage model, will create a fruitful feed-back effect that will improve the performance both of the matching estimation and of the inferential procedure. See also Tancredi and Liseo (2015) for some preliminary results in the regression context.
Finally note that small area estimation with linked data is still an almost unexplored area of research. Hence the proposed activity has a very high potential to advance knowledge within the specific field of statistical inference with linked data. In addition, the possibility to estimate small area models with linked data has an important impact for official statistics institutes which, by the help of proposed techniques, may produce new small area estimates with the consequent societal benefits.
References
Belin, T. and Rubin, D. (1995). A method for calibrating false - match rates in record linkage. Journal of the American Statistical Association, 90: 694-707.
Briscolini, D., Di Consiglio, L., Liseo, B., Tancredi, A., and Tuoto, T. (2018). New methods for Small Area Estimation with Linkage Uncertainty. International Journal of Approximate Reasoning
B Chen, A Shrivastava, RC Steorts (2017) Unique Entity Estimation with Application to the Syrian Conflict arXiv preprint arXiv:1710.026904
Copas, J. and Hilton, F. (1990). Record linkage: statistical models for matching computer records. Journal of the Royal Statistical Society, A, 153: 287-320
De Blasi, P., S. Favaro, A. Lijoi, R. Mena, Prunster, and M. Ruggiero (2015). Are Gibbs-Type Priors the Most Natural Generalization of the Dirichlet Process? IEEE Tranactions on Pattern Analysis and Machine Intelligence 37,2, 803¿821
Fortini, M., Liseo, B., Nuccitelli, A., and Scanu, M. (2001). On Bayesian record linkage. Research in Official Statistics, 4: 185-198.
Jaro, M. (1989). Advances in record-linkage methodology as applied to matching the 1985 census of Tampa, Florida. Journal of the American Statistical Association, 84: 414-420.
Kim, G. , Chambers, R. (2012). Regression analysis under incomplete linkage. Computational Statistics & Data Analysis 56 2756¿2770.
Lahiri, P. and M. D. Larsen (2005). Regression analysis with linked data. Journal of the American Statistical Association 100, 222-230
Larsen, M. (2005). Advances in Record Linkage Theory: Hierarchical Bayesian Record Linkage Theory. Proceedings of the Section on Survey Research Methods, American Statistical Association, 3277-3283
Larsen, M. D. and Rubin, D. (2001). Iterative automated record linkage using mixture models. Journal of the American Statistical Association, 96: 32-41.
Liseo, B. Tancredi, A. (2011). Bayesian estimation of population size via linkage of multivariate normal data sets. Journal of Official Statistics 27, 491¿505
Sadinle, M. and Fienberg, S. E. (2013). A generalized Fellegi-Sunter framework for multiple record linkage with application to homicide record systems. Journal of the American Statistical Association, 108: 385-397.
Sadinle, M. (2014). Detecting duplicates in a homicide registry using a Bayesian partitioning approach. The Annals of Applied Statistics, 8(4): 2404-2434.
Sadinle, M (2017). Bayesian Estimation of Bipartite Matchings for Record Linkage. Journal of the American Statistical Association
Steorts, R. C. (2015). Entity Resolution with Empirically Motivated Priors. Bayesian Analysis, 10: 849-875.
Steorts, R. C., Hall, R., and Fienberg, S. E. (2016) A Bayesian approach to graphical record linkage and de-duplication. Journal of the American Statistical Association. 111.
A. Tancredi, B. Liseo (2015), Regression analysis with linked data: problems and possible solutions, Statistica 75 (2015) 19¿35.
Tancredi, A. and Liseo, B. (2011). A hierarchical Bayesian approach to record linkage and population size problems. Annals of Applied Statistics, 5: 1553-1585