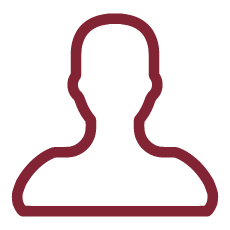
Applications to medical data of analysis techniques developed in Particle Physics are more and more frequent. Among these, Artificial Intelligence (AI) methods, and Deep Learning (DL) in particular, have proven to be extremely successful for a wide range of application areas including medical ones. However, their "black box" nature is a barrier to its application in clinical practice where interpretability is essential. To this aim, we propose to quantify strengths and to highlight, and possibly solve, weakness of the available explainable AI methods in different applicative contexts. Indeed, one aspect hindering so far substantial progress towards explainability AI (xAI) is the fact that usually the proposed solution to be effective needs to be tailored to the specific applications, and it is not easily transferred to other domains. In ADeLE we will test the same array of xAI techniques to several use-cases, intentionally chosen to be heterogeneous with respect to the types of data, learning tasks, and scientific questions to find, as much as possible, general solutions. We will benchmark the xAI algorithms on the segmentation of public available brain images, then, we will apply them to the selected use cases, which are: the estimation of the probability of Spread Through Air Spaces (STAS) in pulmonary adenocarcinoma patients to personalize the treatment suggesting in advance the surgical resection depth; improving the emulation of nuclear reaction models of interest for ion-therapy; and finally to explore the possibility of improving the diagnosis of pulmonary diseases, such as the one caused by COVID-19, using ultrasound.
With the ever-increasing use of AI in many fields, the interpretability of AI output becomes one of the urgent needs in the field. The efforts in improving AI explainability have focused over the last years across three main areas. Firstly, the development of tools and techniques to visualize in a quantitative fashion the way information is processed in their adaptable layers, moving from a purely black-box implementation to what has been defined as a "grey-box" model. These efforts have concentrated mainly on computer vision applications, through the development of visual maps and atlases [1]. Secondly, the design of methods to explain a single prediction of a network, in general by highlighting part of an image [2] or a text [3] that have a strong positive or negative impact on the prediction itself. Finally, a more limited number of authors has tried to complement the networks with globally interpretable explanations by, e.g., model distillation [4]. These works have been applied mostly on natural images and texts (such as the ImageNet database and Wikipedia). Their successful use in the cases described in the previous section raises several fundamental challenges, ranging from the scalability of these algorithms to high-dimensional data to the need for certifiable guarantees on the explanation itself and development of interfaces for non-expert users. Within ADeLE we will:
· select an array of xAI techniques; provide methods to integrate this array into new and existing AI systems;
· develop new explainability methods, including them in the array of xAI methods;
· test and evaluate the effectiveness of the xAI array of techniques on the selected use-cases described in the previous section
Moreover, new opportunities and applications fostered through explainable AI will be identified from the results of the methods applied to the different use-cases.
ADeLE is extremely interdisciplinary from both the topics covered and the skills and knowledge of the different participants. ADeLE will have a great impact on the selected use cases that can be quantified in terms of scientific publications as well as skills developments, in particular training of young researchers and doctoral level students. To be more specific:
· UC1 the stratification of lung adenocarcinoma patients prognosing STAS before surgery would be a step forward in the treatment personalization for these patients and would solve an unmet clinical need;
· UC2 emulating a state-of-the-art theoretical model for low energy nuclear interactions would improve enormously the precision of MC simulation of ion-therapy without having its running overhead;
· UC3 LUS could effectively be used as a fast tool in the diagnosis of interstitial pneumonia, as the one that COVID-19 causes, and monitoring the disease progress, however its sensitivity is strongly dependent on the operator's skill. We aim at mitigating this dependency with a xAI algorithm. Moreover, we intend to evaluate the pulmonary involvement extracting from the image a quantitative estimation of the fluid percentage per each portion of the lung
[1] M.D. Zeiler, and R. Fergus, 2014. Visualizing and understanding convolutional networks. In European conference on computer vision (pp. 818-833). Springer.
[2] M. Sundararajan, A. Taly, and Q. Yan, 2017. Axiomatic attribution for deep networks. In Proceedings of the 34th International Conference on Machine Learning-Volume 70 (pp. 3319-3328). JMLR. org.
[3] A. Vellido, 2019. The importance of interpretability and visualization in machine learning for applications in medicine and health care. Neural Computing and Applications, pp.1-15.
[4] A. Dhurandhar et al., 2018. Improving simple models with confidence profiles. In Advances in Neural Information Processing Systems (pp. 10296-10306).