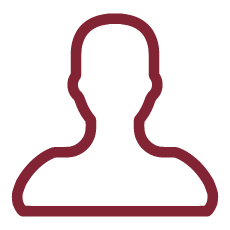
Background: Glioblastoma Multiforme (GBM) is the most lethal primary brain tumor of the adult, with dismissal overall survival. Patient genetics play a crucial role in planning therapeutic intervention, with demonstrated effects on survival. This includes well-known biomarkers such as MGMT or IDH, but also GLUT1 and 3, which may be targeted by future therapies to restore a more normal metabolism in GBM. The gold-standard procedure for histologic and genetic diagnosis of GBM is pathological sampling through brain biopsy or surgery. Along with the risk for complications, high costs and misinterpretation rate, biopsy-based methods may face incomplete sampling due to spatial heterogeneity of GBMs. Artificial intelligence may help overcoming these drawbacks, by providing non-invasive reliable and reproducible predictive models for patient outcome.
Aim: Our aim is to build an artificial intelligence model predictive for the diagnosis of GBM, molecular type and a comprehensive panel of genetic alterations, including MGMT promoter methylation, IDH mutation, EGFR amplification, P53 mutation, Ki-67 status, ATRX loss and GLUT 1/3 expression. We intend to build this model from MR data and digitalized histology slides, by employing machine learning and deep learning techniques.
Methods: We will extract radiomic features from three volumes of interest MR images of GBM: enhancing tumor, non-enhancing tumor and necrosis. We will extract deep features from digitalized histology slides by means of a convoluted neural network. After feature selection, data will be fed to multiple machine learning classifiers to achieve best performance.
The accuracy of the above mentioned studies is far from perfect, and an attempt of building a predictive model for patient survival and all the genetic alterations of GBM has never been pursued before. Particularly, novel biomarkers expression, such as GLUT 1/3 has never been evaluated by radiomics. One of the reasons for the sub-optimal performance of radiomic models is limited patient samples and lack of data diversification. The addiction of pathologic data from digitalized histologic slides might overcome this limitation and boost model performance.
In fact, tissue architecture investigated by pathology has well-known correlates on MR imaging. Several studies have validated the correlation between multiparametric MR and histology, including correlation between diffusion-weighted imaging and cellularity [Laviolette et al., 2014; Chang et al., 2017], perfusion-weighted imaging and vascularity [Jain et al., 2015; Nguyen et al., 2016], water content and T1/T2 relaxation times [Ngo et al., 1985; Naruse et al., 1986]. Different tumoral components are characterized by specific parameters, such as perfusion-based for enhancing tissue and diffusion-based for peri-tumoral edema [Barajas et al., 2012]. Furthermore, tumor genetics has a structural correlate in the tissue [Belden et al., 2011], so that imaging-based habitats may reflect tumor signaling pathways [Dextraze et al., 2017]. Few studies have reported a correlation between highly predictive radiomic features and pathologic evidence from tailored biopsies. Hu et al. reported the correlation between platelet-derived growth factor receptor (PDGFRA), EGFR, cyclin-dependent kinase inhibitor 2A (CDKN2A), retinoblastoma 1 (RB1) and several radiomic features, mainly texture-based [Hu et al., 2016]. These evidence support the idea that an integrated model merging MR and pathology data would show improved performance.