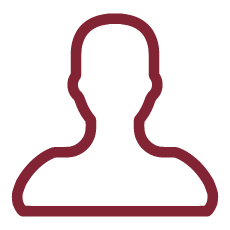
This project deals with data ranking, preference and ordinal data analysis, which is a theme of peculiar relevance in applied sciences. We will provide new methodological devices for treating ranked data and discuss alsotheir applications in important empirical contexts.
Under a methodological perspective, two main directions are explored. By one side, we adopt a model free approach and develop new clustering procedures. The employed techniques include fuzzy clustering, discrete copulas and Bayesian networks; by the other side, we discuss rank-size problems by identifying best fit curves approximating ranked data. Under this perspective, the project aims at developing new fitting law and synthetic indicators for ranked data. The theme of the assessment of the outliers will be also faced with specific attention.
Patr of the project will be devoted to the identification of real data applications of peculiar meaningfulness. Among them, the project aims at analysing the yearly official data at provincial level that Istat produces on the quality of life and socio-economic well-being indicators of the italian population.
The research project is expected to add to the knowledge of the ranking procedures but also to the considered empirical instances. In terms of methods, we aim at creating new versatile instruments that can be suitably adopted in the broad context of statistical analysis of the data. Under the point of view of the application, we pursue the ambitious target of contributing to a deeper understanding of the explored socio-economic phenomena; in so doing, we aim at providing also instruments whose informative content can be of real usefulness for policymakers.
The research project is expected to increase the knowledge of the ranking procedures ¿by both sides of methods and applications- in several respects.
The use of the Fuzzy C-Medoids technique to detect clusters of subjects with similar preferences patterns ¿which is already a novel technique at the frontier of applied research- could be efficiently extended to account for high levels of complexity and to face important issues.
Moreover, beyond the advantages provided by the joint use of discrete copulas and fuzzy techniques, we argue that the definition of a new measure of dependence for ordinal variables based on discrete copulas could be of interest for classification purposes and also for a broad class of methods and models concerning ordinal variables.
The extension of the Fuzzy C-Medoids algorithm to multivariate preference data observed on several different occasions or according to different criteria is also a value added of the project. The challenge is to define a suitable metric on items, rather than on judges, satisfying all properties of the distances.
Importantly, another innovative aspect of the project lies in the application of new structural learning techniques for discrete BNs in the context of ordinal\ranked variables; such devices will be exploited and applied to real datasets.
Further innovative aspects of the projects can be identified by looking at the rank-size analysis. Indeed, the employment of the rank-size theory allows to build a unified macro system ¿which is described by the best fit curve- by starting from microscopic observation of the ranked values of a given phenomenon. By the exploration of the shape of the best fit curve, we expect to gain relevant information on the investigated sample and on its future outcomes.
The identification of new classes of rank-size laws is expected to foster the representation of peculiar characteristics of the relationships between ranks and sizes. In this respect, new socio-economic insights are expected from the conceptualization of new synthetic indicators. The informative content of the considered indicators depends strongly on the phenomenon under scrutiny; under this perspective, testing the rank-size regularities over empirical instances coming from a wide range of socio-economic contexts could be of effective usefulness also by the viewpoint of the policymakers.
References (see also the previous Section)
[MM1] Murphy, T.B. & Martin, D. (2003) Mixtures of distance-based models for ranking data. Computational statistics & Data Analysis 41(3-4), 645-655
[MM2] McBratney, A. & Moore, A. (1985). Application of fuzzy sets to climatic classification. Agricultural and Forest Meteorology 35(1-4), 165-185
[MMDMMC] Martínez-Mekler, G., Martínez, R. A., del Río, M. B., Mansilla, R., Miramontes, P., & Cocho, G. (2009). Universality of rank-ordering distributions in the arts and sciences. PLoS ONE 4(3).
[MT] Mollica, C. & Tardella, L. (2017). Bayesian plackett-luce mixture models for partially ranked data. Psychometrika 82(2), 442-458
[OP] Ottaviano, G. I., & Puga, D. (1998). Agglomeration in the global economy: a survey of the `new economic geography¿. World Economy 21(6), 707-731
[PT] Pavan, M., & Todeschini, R. (2008). Scientific data ranking methods: theory and applications. Elsevier
[S] Smith, B.B. (1950). Discussion of professor Ross¿s paper. Journal of the Royal Statistical Society B 12(1), 41-59
[T] Thurstone, L.L. (1927). A law of comparative judgment. Psychological Review 34(4), 273
[WK] Wedel, M. & Kamakura, W. (1998). Market segmentation: Conceptual and me-thodological foundations. SKluwer Academic Press, Boston
[Z] Zipf, G., (1949). Human Behavior and the Principle of Least Effort, Cambridge, MA: Addison-Wesley Press