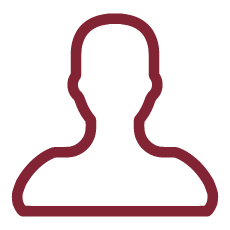
Quantitative understanding of behaviour of living organisms is a topic of great importance in biophysical literature. In particular, interest has arisen around interacting micro-swimmers, which is an umbrella term for small aquatic organisms, from bacteria to small animals. Recent advances in reinforcement learning, a form of machine learning, allow for more than descriptive analysis and open the possibility of probing how certain behaviour can emerge as optimal strategies of self interested agents.
In this work, we focus on prey-predator interactions in low-Reynolds hydrodynamics. We assume agents can can only receive information via hydrodynamical sensing and play a zero sum game: while the predator purpose is to catch the prey, the prey has to avoid encounters. Since both agents have to rely on incomplete information, trivial strategies are not available in principle. We intend to apply a non-black box machine learning algorithm in order to be able to get insight both in the strategies and how hydrodynamical information is relevant.
While most statistical physics of active particles is aimed at understanding collective behaviour in a cooperative or at least non adversarial way, this work explores the role of conflicting interests between agents. This possibility is only available thanks to tools which have been recently introduced and which are opening new perspectives on the study of intelligent biological behaviour.
The combination of hydrodynamics interaction and intelligence in this framework is a key point. Since agents are not omniscient but must infer their knowledge of the world from the environment, our problem can be classified under the umbrella category of partial observability learning [1]. Our peculiar design of the observable quantities is intended to overcome the shortcoming of black box approaches to learning, so that we intend to infer how the agents are capable of making sense of the signals they detect. In this way, our study can go beyond a mere observational approach, allowing to reverse engineering optimal strategies chosen by the agents. Hence, our understanding of the problem does not have to be limited to the specific problem, but can be reframed in a more general context. While there exist several descriptions of how animals behave in their environment, it is often unclear why specific behaviours emerge or what kind of information is relevant to them. A picture that is emerging, to which we intend to contribute, is that organisms can display relatively complex strategies with simple modelling (see e.g. [2])
By combining the previous points, we aim at contributing to the emerging field of quantitative description of intelligent behaviours. This field is of great interest for both theoretical and applicative purposes, but a gap still exists between understandable but otherwise low-complexity applications and high performance real world scenarios which still rely on black box methods, such as deep neural networks. Our problem is simple enough to allow for reproducibility, statistical analysis and physical insight, while being at the same time sophisticated enough to allow for non-trivial behaviours to emerge.
[1] Jaakkola, T., Singh, S. P., & Jordan, M. I. (1995). Reinforcement learning algorithm for partially observable Markov decision problems. In Advances in neural information processing systems (pp. 345-352).
[2] Mazzolini, A., & Celani, A. (2020). Generosity, selfishness and exploitation as optimal greedy strategies for resource sharing. Journal of theoretical biology, 485, 110041.