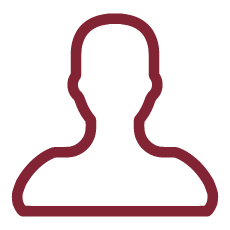
Analysis of 3D data is a field that is increasing its importance in the computer science community due to the great diffusion and wide availability of devices that enable everyone to acquire and generate 3D data autonomously. In the last decade, the amount of available 3D data grows impressively and nowadays, many tools for the analysis, generation and manipulation of this kind of data come to light. Simultaneously, the computer science community proposed several frameworks and tools based on deep learning and artificial intelligence achieving high performances in challenging tasks. With this proposal, we aim to merge these topics to generate new instruments for targeting applications on 3D data. The kind of applications that we will target in this project goes from the shape correspondences to the generation and the analysis of collections of surfaces. The availability of 3D data is, at the same time, a great motivation to develop new tools and a necessary condition to define our instruments on data-driven approaches. To be more concrete, the principal objective of this project is to study and analyze different ways to learn a latent encoding for 3D data that enable us to solve for independent tasks exploiting a unique representation or to define additional constraints for the learning to address a specific application. The recent advances in the context of latent space definition, disentanglement and 3D shape generation will be the base of our work. We plan to develop new techniques that could give rise to outstanding results in challenging scenarios enabling new applications.
This project is new in several aspects. First, it will generate new tools for the analysis of shape collections. Secondly, it will give rise to a natural context where learning strategies could help to improve the performance of state-of-the-art methods. Finally, learning a latent representation will provide us with a new possible shapes parametrization that could be injected into existing pipelines paving the way for new applications and outstanding results.
More concretely, in the context of analysis of shapes collections, we will mainly focus on the medical context where a huge amount of data have been recently generated and that naturally offer several real application with a strong impact in the everyday life. AT the moment there are several works from the team of Maks Ovsjanikov and Mirela Ben-Chen on this topic.
Starting from the paper "Map-Based Exploration of Intrinsic Shape Differences and Variability" by Rustamov et al. published at SIGGRAPH 2013, where based on the framework of the functional map they proposed an operator (the shape difference operator) to analyze the variations between shapes in a collection, they independently develop their tools for this specific task.
M. Ben-Chen then studied this problem in "Cross-Collection Map Inference by Intrinsic Alignment of Shape Spaces" published in CGF 2014, and then in "Robust Shape Collection Matching and Correspondence from Shape Differences" recently published in CGF 2020. M. Ovsjanikov proposed some improvements in the more recent papers: "On the Stability of Functional Maps and Shape Difference Operators" published in CGF 2017, "Limit Shapes: A Tool for Understanding Shape Differences and Variability in 3D Model Collections" published in CGF 2019 and "Consistent ZoomOut: Efficient Spectral Map Synchronization" published in CGF 2020. In all these papers, the main idea is to define a correspondence between all the pairs of shapes in the collection and then exploit these correspondences to define a parametric space where the differences between objects could be better analyzed and studied. The methods listed above are all based or related to the framework of the functional map and do not involve any data-driven solution or learning-based approach. This is the space where we want to place our contribution, closing the gap between existing solutions and the learning techniques. In particular, M. Ovsjanikov recently proposed "Geometric analysis of shape variability of lower jaws of prehistoric humans" published in the Journal L'Anthropologie in 2020, where the tools developed in the manuscripts listed above are used to analyze the shape variability of lower jaws of prehistoric humans. This paper inspired our project and could be seen as a sketch of what we aim to produce. The main differences are that: I) we want to involve learning procedure in our pipeline; ii) we want to target specific problem from the medical scenario analyzing the anatomical components of the human bodies providing the necessary level of accuracy necessary for the context. Furthermore, we aim to work more on the learning of a latent space that could allow us to have a detailed representation of the shapes in our collection and at the same time to be suitable for analysis and generation of new objects. This aspect is strongly related to the recent work we develop in the GLADIA team and that was recently published at the ICML 2021 conference. In our paper "Learning disentangled representations via product manifold projection". In this paper, to which we refer for all the related literature, we proposed a novel technique to learn a latent space where the different factors that are involved in the generation of the data involved in the collections could be separated as orthogonal directions in the latent space. Our method provides a space that can be easily explored, controlling the different factors that change along different paths defined in the latent space. Building on this theory we aim to find a new latent space definition that could be used for the exploration of 3D data collections for several applications: shape generation, retrieval, clustering, anomaly detection among many others.