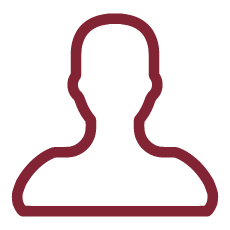
Artificial intelligence (AI) methods and machine learning (ML) are receiving growing attention given their potential impact on diverse areas of life sciences. In the forensic field, ML techniques have been mainly used in the area of Neuroprediction, with the raising of some ethical and legal concerns regarding their questionable fairness, accountability and transparency. Although algorithmic risk assessments can be perceived as a means to overcome human bias, they often reflect prejudice and institutionalized bias, as AI is generally trained on data that may themselves reflect evaluators¿ biases. The majority of studies conducted on this topic so far failed to isolate precisely which factors may be causing the bias, probably due to their naturalistic designs.
To overcome these ethical issues, this highly interdisciplinary project aims at extending a serious gaming¿based software platform (developed in a previous project) able to learn forensic decision strategies and identify cognitive biases via synthetic cases (Virtual Defendants).
Our unique team comprises forensic psychiatrists, coroners and a computer scientist PhD student (plus two senior AI experts, Mancini, and Tronci, as external members), and allows us to exploit:
1) Retrospective data available in 500 forensic psychiatric reports made in criminal proceedings
2) Expert knowledge for all the relevant forensic domains. Expertise on forensic experts¿ decision making and insanity defense will be covered by a post-doc position.
3) Acquire further Expert Knowledge through Synthetic cases (Virtual Defendants) generated by our AI-powered serious gaming platform
Our envisioned computational approach builds on our experience in this area matured with the coordination of the PAEON FP7 project (paeon.di.uniroma1.it ¿ PI: Tronci; Key person: Mancini), where computerized decision strategies for complex protocols for assisted reproduction have been formalised and offered to practitioners within a decision support system.
To date, AI techniques in healthcare have been mainly applied for the diagnosis, prognosis, treatment prediction, and the detection and monitoring of potential biomarkers.
We believe that Forensic Psychiatry, and specifically our proposed study of forensic experts¿ cognitive biases through the use of a serious gaming¿based software platform (where forensic expert will be involved as players), could be a novel groundbreaking application of AI in such a safety-critical area. In particular, by providing synthetic cases (Virtual defendants) to the players (our forensic experts), intelligently generated by our AI, it will allow us to overcome the limits linked to the study of naturalistic settings and the limits linked to the already existing algorithms which have been developed and trained on retrospective data. In fact, although retrospective data such as forensic psychiatric evaluations may be important for the study of forensic evaluators¿ decisional processes, they are usually not enough to learn correct general decision strategies that cover all situations and to clearly underline cognitive biases. This is because available retrospective data are usually limited, often do not log all the aspects of the case that impacted the decisions, and, virtually always, they represent only a fraction of the whole spectrum of variability that the decision makers might face in reality. This is especially true for atypical cases (which are often poorly represented in retrospective data), where the availability of a qualified DSS would indeed bring the greatest advantages. In addition, retrospective data themselves are not free from biases. This is an issue of uttermost importance since most of the algorithms used in the forensic field are developed and trained on the bases of such data. For example, one of the most famous case of supposed AI prejudice regards COMPAS, an algorithm widely used in the US to guide sentencing by predicting the likelihood of a criminal reoffending, which turned out to be racially biased against black defendants (27) and to be a ¿sexist algorithm¿ because its algorithmic outcomes seem to systemically overclassify women in higher-risk groups (28).
Differently from these previous approaches, our serious gaming software platform will allow us to extend the identification of cognitive biases and the decision strategy learning process also towards atypical cases of the spectrum of possible scenarios, and to improve the internal consistency of the learnt decisions.
This research project represents the first step in shedding light on forensic evaluators¿ decisional processes and cognitive biases during the evaluations regarding criminal responsibility and social dangerousness, in order to improve their reliability and validity. We deem, in fact, that increasing awareness and trying to minimize the impact of cognitive bias could lead to a more accurate approach in insanity evaluation and thus result in an improvement in the whole criminal justice process.