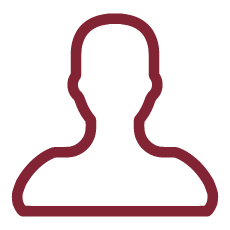
The problem of recognizing and therefore distinguishing different audio sources is an easy problem for humans: recognizing the various instruments in a song and what they are playing is a simple task even for an untrained ear, as well as concentrating on a person speaking during a dinner or an event full of people.
Recognizing and identifying different audio sources present within a mixture of signals is a relatively accessible task even for a shallow neural network, but isolating the individual sources and hence separating the different contributions is not easy at all, even for an advanced neural architecture.
This project aims to elaborate and train an innovative deep neural network capable of separating a mixture of audio signals even very different from each other, in order to obtain a clear and clean separation of the various components of the raw signal, exploiting a new class of machine learning frameworks called generative adversarial network.
This approach has been widely used in the generative field to create new and original audio signals (such as songs or people's voices) starting from existing samples, but has never been used to solve this separation problem and I strongly believe that this method, applied to this kind of problem, will lead to a new state of the art in the field of audio separation.
The innovation of this research consists in the introduction of generative methods to the already promising separation system described above which operates directly in the time domain.
Deep generative models are a way of learning any type of data distribution and are already widely successfully used in computer vision and computer graphics to generate new examples never seen before, sampled from a distribution learned from the network by observing the input data.
As regards the audio sector, WaveNet, developed by Google, is one of the most encouraging human voice generation models ever heard, which generates realistic-sounding human-like voices with all the nuances that characterize the human voice. Jukebox and MuseNet, two deep neural networks developed by OpenAI, represent the frontier as far as music generation is concerned. They are capable of generating music, including elementary singing, as raw audio in a variety of genres and styles of artists or of creating musical compositions by combining very different styles.
Using this type of neural network to solve the signal separation problem could represent a turning point in the field of audio processing, since levels of accuracy never reached before could be achieved and therefore this could open a new path for research in this area.
In this project, the generative act would replace the learning process of the masks to be applied to the original raw signal. In fact, the neural network would listen to the audio signal and sample, from a previously learned musical instruments distribution, the various contributions that make up the piece of music, recreating note after note all the instruments of the track, just like a human would do.