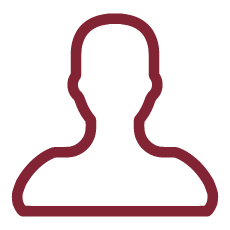
The quantity of data available to data-holders institutions has been rapidly increasing in recent years. A notable example is the sheer number of administrative sources with individual-level information that become available to national statistical institutes. This new asset of data required a shift of focus to new specific methodologies for statistical analysis in official statistics. A family of models has received renewed attention in this setting, namely the latent class models.
These models are used in various stages of the statistical production with different goals but, in general, they share a common perspective: since these kinds of data are not directly collected for statistical purposes, the information required by the statistician is not perfectly aligned with the information available. As a consequence, we exploit the information redundancy coming from the integration of multiple sources targeting the same units/variables. In this approach, the latent classes are used to model the desired classification of the units.
Examples of applications include every phase of the statistical treatment: from handling record-linkage errors, to editing and imputation, to estimation of the size of a target population.
Latent class models belongs to the wider class of finite mixture models and thus are particularly appreciated for the easiness of use and flexibility, and generated countless extensions in literature. In this project we propose to define and compare different Bayesian approaches to this class, applied to the listed practical context, and to develop a Bayesian procedure to facilitate model selection within the class.
As an additional source of uncertainty, model selection appears to be crucial for the family of LCM. Literature on model selection for LCM focuses almost exclusively on the choice of the number of latent classes in order to balance goodness of fit and parsimony in the number of parameters. However, in our applications, where the number of latent classes is fixed by the assumption of the analysis, we lack a specific methodology for model selection. In all application we wish to study, the classic conditional dependence assumption appears to be too simplistic and we usually refer to a class of log-linear LCM. Model selection within this class appears to be far from straightforward. The contributions in this respect focus on detecting violation of the conditional independence assumption by looking for pairwise dependencies between manifest variables (e,g., [1] [2]). However, we are willing to consider more complicated dependencies, and the number of possible models increases dramatically with the number of observed variables, making the process impractically time-consuming. We think that a reliable procedure to deal with model selection in a more automatic way would certainly be of interest.
A full Bayesian approach would allow the introduction of a probability measure over the set of models we want to consider, and evaluate the posterior probability of each model with a single procedure. In capture-recapture applications, instead of simply estimating the posterior probabilities for model selection, we plan to implement model-averaging techniques and evaluate the posterior distribution of the population size, marginalized over the set of possible models.
Model-averaging techniques have been explored in machine learning literature to select the variables in LCM under the conditional independence assumption, but, to the best of our knowledge, have never been proposed for more complex models of this kind.
1. Oberski, D.L., van Kollenburg, G.H., and Vermunt, J.K., 2013. A Monte Carlo evaluation of three methods to detect local dependence in binary data latent class models. Advances in Data Analysis and Classification 7.3: 267-279.
2. Qu, Y., Ming T:, and Kutner, M., 1996. Random effects models in latent class analysis for evaluating accuracy of diagnostic tests. Biometrics 797-810.