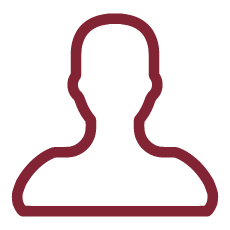
In this project we consider the problem of sample size determination (SSD) under a Bayesian framework. We focus on experiments in which multiple priors are available that formalize pre-experimental information and uncertainty on model parameters. In this setup, prior-to-posterior analysis can lead to discordant final inferential conclusions.
In a recent contribution, Joseph and Belisle (2019) proposed a measure of discrepancy between credible intervals based on two conflicting priors and defined a sample size criterion aimed at guaranteeing posterior consensus between them. In this sense, this criterion ignores the achievement of success of the trial, that is usually thought in terms of evidence.
Our proposal is to introduce a method for SSD that simultaneously control the achievement of minimal evidence and consensus, measured by appropriate functions of the posterior distributions. In some preliminary works we have started considering efficacy and superiority trials involving binary outcomes.
The goal of the present project is to elaborate on this idea and to widen the scope of the existing methodology in several directions according to the following tasks.
TASK 1. Providing explicit and general results for trials with different goals (e.g. equivalence, non-inferiority) and with different outcomes (e.g. continuous, categorical or both, ¿).
TASK 2. Comparing the properties of several SSD criteria (e.g. based on different alternative measures of consensus).
TASK 3. Developing the code for the implementation of the proposed criteria in an R package supplemented with a Shiny app directly accessible to the final users.
As highlighted in the previous sections, to the best of our knowledge, the problem of determining the sample size of a trial by ensuring a pre-posterior joint control of consensus and evidence has not been considered yet. The idea of achieving a posterior agreement between potentially conflicting sources of prior information is interesting and can be accommodated in the Bayesian framework in a straightforward way. However, we are aware that it is not completely satisfying from the point of view of the clinicians who typically assume that the goal of the experiment is clearly stated and, therefore, require a sample size that guarantees a sufficiently large chance of success of the trial.
Hence, our research could contribute to spread the use of Bayesian methods that allow to handle the presence of multiple sources of information and, at the same time, comply the usual way of thinking of experts. This goal will be reachable also thanks to the availability of an R package and of a Shiny app that will be directly usable by practitioners.