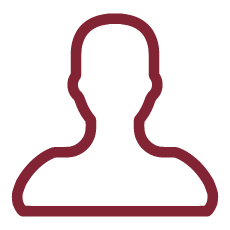
Interim monitoring is widely adopted in clinical trials to minimize patients' exposure to not sufficiently effective therapies. In this project we aim at developing Bayesian monitoring rules for phase II single-arm trials, that allow to account for crucial issues such as, for instance, the uncertainty in the target response rate, the association between efficacy and toxicity data and the presence of more than two decision makers.
Our interest is mainly focused on bivariate binary outcomes, that represent efficacy and toxicity of the experimental treatment. Most of the Bayesian monitoring rules previously proposed are based on posterior probabilities and exploit marginal models for the two endpoints. We instead suggest to use the joint Dirichlet posterior model to define the experimental treatment as successful, in order to account for the association between efficacy and toxicity. We also aim at evaluating the performance of Bayesian decision rules based on different early stopping boundaries for the posterior probability of interest. As an alternative approach, we will exploit predictive probabilities for interim monitoring of efficacy and toxicity endpoints. The idea is to construct stopping rules based on the predictive probability that the trial will yield a positive outcome, if it continues to the planned end. All these Bayesian methods will be evaluated and compared with already existing procedures in terms of frequentist operating characteristics.
A further purpose of our research group is to adapt the sequential trial monitoring mechanism to the formal framework of a decision-theoretic approach, under the assumption that analysis and design are performed by multiple parties. In this context, the focus is on the construction of a predictive measure of the conflict between their opposite choices. Based on this measure, we aim at establishing stopping rules expressed in terms of the possibility/impossibility of bringing a consensus between the two parties.
The participants of this project have a consolidate and multi-year experience in the area of experimental design and in the methodological research related to clinical trials. Hence, we expect to provide innovative contributions from a methodological point of view in relation to the following tasks that schematically summarize the main objectives of the project.
TASK 1. BAYESIAN MONITORING RULES FOR BIVARIATE BINARY OUTCOMES BASED ON POSTERIOR PROBABILITIES
We aim at expanding the already presented procedures to monitor bivariate binary outcomes through posterior probabilities in two directions as follows.
(a) Instead of considering marginal models, we will exploit the joint Dirichlet model to define the trial success, in order to involve the association between the two endpoints in the decision rules.
(b) We will propose new monitoring strategies that compare the posterior probabilities of interest with monotonically increasing functions with n/N, avoiding the use of fixed cut-off values, in order to have decision rules that differ according to the amount of information accumulated at the interim look.
TASK 2. BAYESIAN MONITORING RULES FOR BIVARIATE BINARY OUTCOMES BASED ON PREDICTIVE PROBABILITIES
The idea of monitoring both efficacy and toxicity endpoints in phase II studies through the use of Bayesian predictive probabilities to the best of our knowledge is new. Our purpose is to develop Bayesian rules based on this approach, that has the advantage of representing a compromise between the current information and the future sample size. We also believe that the predictive approach is particularly appealing for monitoring bivariate binary outcomes because it allows to account for the association between the two endpoints observed ad interim, even when the treatment success is defined through marginal models.
Besides providing Bayesian decision rules, we also aim at innovating the current understanding by investigating different procedures to determine the optimal value of the total sample size N by ensuring good frequentist operating characteristics. Furthermore, the interest will be focused on the use of informative Dirichlet prior distributions, in order to represent archetypal points of view that may be used to cautiously monitor the trial.
TASK 3. ACCOUNTING FOR UNCENTAINTY IN THE RESPONSE RATE OF THE STANDARD TREATMENT
A critical issue in phase II single-arm studies is the failure to acknowledge any inherent variability in the historically estimated response rate of the standard treatment. In fact, the response rate of the experimental agent is typically compared with a fixed target value. Following Matano and Sambucini (2016), we aim at addressing one of the major concerns about the use of historical controls, i.e. the potential bias in the comparison, by extending the predictive design of Lee and Liu (2008) to incorporate uncertainty in the response rate of the standard treatment through the use of prior distributions.
TASK 4. SEQUENTIAL TRIAL MONITORING BASED ON THE PREDICTIVE CONTROL OF THE CONFLICT BETWEEN ADVERSARY DECISION-MAKERS
Our purpose is to investigate the possibility of adapting the sequential trial monitoring mechanism into the formal framework of a decision-theoretic approach. More specifically we are interested in the peculiar context of adversarial decision-making: we assume that two experimenters coexist and we focus on a predictive measure of the conflict between their opposite choices. Based on this measure, we aim at establishing suitable stopping rules expressed in terms of the possibility/impossibility of bringing a consensus between the two parties.
TASK 5. DEVELOPMENT OF SOFTWARE TOOLS TO IMPLEMENT BAYESIAN MONITORING RULES
From the computational point of view, our goal is to provide a systematic implementation of the proposed methods in order to make the software tools available and easily accessible even for the practitioners. In particular the choice of the open source software R will allow us to share our contribution with a wide research community, guaranteeing flexibility, reproducibility and transparency.
REFERENCES
* Lee JJ and Liu DD (2008). A predictive probability design for phase II cancer clinical trials. Clinical Trials, 5(2), 93-106.
* Matano F, Sambucini V (2016). Accounting for uncertainty in the historical response rate of the standard treatment in single-arm two-stage designs based on Bayesian power functions. Pharmaceutical Statistics,15(6), 517-530.