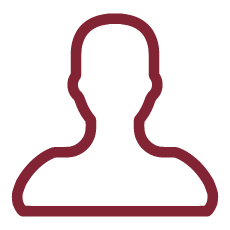
Business Process Management (BPM) is a central element of today organizations. However, in the era of Big Data and Internet-of-Things, all real-world domains are becoming cyber-physical (e.g., consider the shift from traditional manufacturing to Industry 4.0), with the presence of connected artifacts (such as sensors and smartphones) producing huge amounts of data and events that dramatically influence the enactment of business processes (BPs). In such unconstrained settings, BPM professionals lack the needed knowledge to model all possible contingencies and exception handlers at the outset. Consequently, any running BP will be inevitably affected by an increasing number of unplanned exceptional situations, and thus will always has knowledge gaps that prevent it to adapt to novel situations. To overcome this issue, BPbots will develop a new breed of intelligent techniques that go beyond traditional preprogrammed solutions in BPM and enable BPs to act autonomously in presence of unpredictable environments. The philosophy of BPbots is not to re-design or change existing BPs, but to make them evolve as intelligent entities able to automatically adapt to new situations never encountered before, without the need of any human intervention. The use of action-based formalisms in Artificial Intelligence (AI) will be the key to harness and interpret the ever-changing knowledge of cyber-physical domains and to adapt BPs preserving their base structure. Remarkable results obtained by the applicant in Automated Planning in AI for solving challenging problems of process adaption and mining provide evidence of the effectiveness of AI technologies being deployed alongside BPM, and put the applicant in a strong position to realize this project. BPbots will pave the way for ground-breaking scientific and technological advances in the BPM field towards the development of a new generation of BPs able to behave intelligently in any possible situation.
Given the key role that automated mechanisms play in our society, the gain of the shift proposed by BPbots will be highly relevant, as it will allow unleashing the full potential of PA to make BPs act autonomously and intelligently in presence of any possible contextual situation.
In this direction, the BPbots project aims at breaking one of the key assumptions of the BPM philosophy, which is the coupling of the PA logic with the BP logic. While this can be acceptable in working domains where the number of exceptional situations is small and predictable, it poses severe limitations when BPs are executed in cyber-physical domains. Thus, the key novel aspect of BPbots is the strong decoupling of PA mechanisms from the BP models.
To pursue this breakthrough, we identified six challenges that will be addressed by the project:
C1: Context Awareness. In cyber-physical domains, contextual knowledge about the domain, including outcomes provided by BP tasks as well as the occurrence of external events, are the main drivers for triggering PA, as BP progression may be continuously influenced by context changes. Focusing on the control flow only - as traditional PA approaches do - would be insufficient. Hence, a key question is how such contextual knowledge can be represented, updated and monitored in a way that it can be used to adapt BPs.
C2: Fully Automated PA. Existing PA approaches leverage a manual specification of exception handlers at design-time or recovery procedures at run-time, but we have acknowledged that this is not the appropriate way to manage PA in cyber-physical domains. A crucial challenge is to develop general-purpose PA mechanisms that enable BPs to automatically adapt in case of unpredictable exceptional situations, thus requiring no predefined handler for building recovery procedures at run-time.
C3: Collaborative PA. A strong assumption of existing PA techniques is that the adaptation of a BP instance will influence just the structure and the progression of the instance itself, i.e., no other running BP will be affected by the adaptation found. To remove this restriction, a relevant challenge consists of developing a mechanism that allows different running BPs to communicate each other to share their local contextual knowledge, in order to develop more sophisticated collaborative PA mechanisms to adapt several BP instances at the same time.
C4: Opportunistic PA. In cyber-physical domains, the presence of sensors allows to collect both malicious and angelic events. If malicious events often lead to exceptional situations, angelic ones are the result of non-dangerous contextual changes that could be exploited to proactively adapt the running BPs to achieve some business goal in a more convenient way. The challenge is to develop PA mechanisms that consider deviations not only as unwanted situations, but also as a natural and valuable part of the work activity.
C5: Long-term BP Resilience. BP resilience determines the long-term ability of a BP to successfully and robustly deal with adversities. The challenge is to make PA mechanisms resilient-driven, so that the most frequent identified failures at run-time can be incorporated into the BP models, to make their future instantiations more robust to deviations and to mitigate the impact of exceptions.
C6: Configuration of BPbots. Traditional BP modelling efforts are mainly directed to the sole control-flow perspective. With the introduction of BPbots to manage PA, an extra modelling effort is required for their configuration. Since one of the main obstacles in applying new modelling approaches to BPM is the "resistance" of BP designers (which do not want to switch from the traditional way of modelling BPs), the challenge is to overcome such a resistance and reduce the modelling effort for configuring BPbots through the development of user-friendly low-code based visual techniques to facilitate the modelling activity.
The above identified challenges will be tackled through the research program developed for the project (see the previous section). It is worth to notice that the Principal Investigator (PI) has already obtained remarkable scientific results in the use of AI techniques to solve efficiently several complex problems from the BPM field, such as the automated synthesis of process models, the automated adaptation of knowledge-intensive processes and the problem of computing trace alignments in process mining. Moreover, on the topic of BP resilience, the PI published a paper at the prestigious CAiSE'17 conference (Gii-Grin A), where he received the Best Paper Award. The above results, published in top conferences and high-ranked journals, demonstrate the feasibility of the research paradigm delineated by the project, which has the potential not only to push ground-breaking scientific and technological advances in the BPM field, but also of positively influencing the communities of Reasoning about Action and Automated Planning in AI.