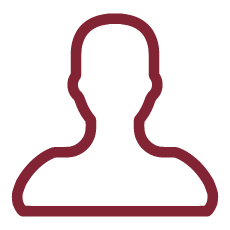
In healthcare daily practice, clinical decisions (e.g., choice of drug and dosage patterns, timing of measurements, etc.) are taken as a consequence of interviews, visual inspections, and clinical assays (e.g., blood samples, RMIs, etc.) on the patient, and aim at achieving given treatment goals, while safeguarding patient¿s health from the possible adverse effects of the chosen treatment. From a control engineering point of view, the physician acts as a controller on the patient (who acts as the plant in a feedback control-loop).
As a result of the standardisation of the clinical workflow, clinical practice has shown that treatment protocols and guidelines improve healthcare quality, and help to avoid risks (for example those related to overlooking routine checks).
Rules prescribed by clinical guidelines, however, are mainly qualitative suggestions for clinical actions that have to be taken facing a concrete clinical situation.
The project aims at supporting the modelling and formalisation of treatment strategies and best practices to be offered within Clinical Decision Support Systems (CDSSs) for different healthcare domains, by using a hybrid approach which combines model checking, gamification, machine learning, active learning, and knowledge engineering techniques.
Namely, the project goal is to design novel general methods and software (hence customisable to several areas of medicine) to:
- Learn core decision strategies from retrospective data from Electronic Health Records (EHRs)
- Acquire and continuously peer-review medical expert knowledge through user-engaging serious games
- Continuously improve the acquired decision strategies by actively learning from intelligently-chosen synthetic medical cases submitted to experts for evaluation.
Thanks to our interdisciplinary team, we plan to evaluate the effectiveness of our methods and software in two areas of psychiatry: mood disorders during pregnancy and forensic psychiatric evaluations.
[Max 5000 chars]
=== Serious games mechanics and user engagement
For our serious gaming platform to be effectively and intensively used by our players, the user interface must be highly usable, and the envisioned game mechanics must be highly engaging. In particular, the involvement, in the validation/refutation of formalised rules, of domain experts with a cultural background very far from mathematics and computer science requires innovative methods to present them rules and constraints in forms, e.g., graphical or narrative, they can immediately understand and modify, if needed.
=== Medical knowledge acquisition via active learning
We will advance the state of the art in active and interactive learning, by providing effective methods for the continuous acquisition and validation (via our serious gaming platform) of medical knowledge by means of independent domain experts.
=== Hybridising machine-learning with knowledge-based approaches
Machine-learning approaches from data are popular nowadays, but suffer in those situations where available training data is scarce, where explainability is a must, and where the presence of biases in the learnt models cannot be tolerated.
In safety-critical settings such as those addressed in this proposal, such weak points of machine learning are amplified. Indeed, gathering massive amounts of retrospective data is extremely difficult in healthcare, and such data are often only partially representative of the whole spectrum of patient peculiarities. Furthermore, lack of explainability of the reasoning that led a CDSS to the provided suggestion hinders the wide adoption of such tools in everyday clinical practice.
We will advance the state of the art in this area by devising methods that allow a sound hybridisation of machine-learning algorithms with knowledge-based approaches, in order to enforce suitable constraints (directly coming from domain knowledge) onto the learnt models and support explainability and biases removal.