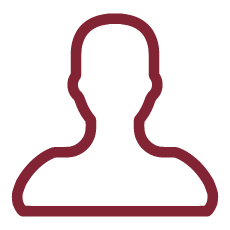
In economics causal analysis is a fundamental instrument to study the effect of a policy or, more in general, the effects of a treatment on an outcome of interest (TE). But, in last decades, researchers put their attention not only on the TEs, but also on causal mechanisms through which this treatment transmits the effects to the outcome. In this case, the researchers want to disentangle the total treatment effect into a direct effect of the treatment on the outcome and into an indirect effect, that operates through one or more intermediate variables, called mediators, that lie in the causal pathway between treatment and outcome. Even in presence of a double randomization of treatment and mediator, these effects are not identified. To tackle this issue, a regression discontinuity approach (RD) is proposed for the identification: in particular, this procedure permits to control for the structural endogeneity of the mediator. A simulation study gives an intuition of these results.
The main contribution of this project is to show the identification and the estimation of the natural direct and indirect effects with an implementation of a Regression Discontinuity design (RDD) to solve the problem of the endogeneity of the mediator. Thanks to the presence of a continuous, observable forcing variable Z that generates variation in the state of the mediator, we can rule out the presence of unobservable and observable post-treatment confounders that jointly affect the mediator and the outcome. In particular, we explain two different models, relying on two different set of assumptions. The first one is less flexible and only a parametric identification is possible, recalling the linear structural equation model of an influential article by Baron and Kenny (1986). In particular in Model 1, Z is affected by treatment and in turn deterministically affects the mediator. In the second model we have a non-parametric identification and then a more flexible framework, in which we don't need the specification of the functional form and, at the same time, the heterogeneity of the effects is allowed. This is due to the fact that Z now is an exogenous variable but still deterministically affects the mediator. In both cases, then, we use a sharp RD, see for instance Trochim (1984), Imbens and Lemieux (2008), Lee (2008), Lee and Lemieux (2010), that permits to have compliers as population of reference.
So, it is the first study that uses RD method to solve the problem of the endogeneity of the mediator and then it is the first methodological contribution that join these two literatures (an intuition about using RD to study indirect effects is given by Angelucci and Di Maro (2010)). Moreover, there are very few studies using quasi-experimental designs: an important contribution is given by E. Deuchert, M. Huber and M. Schelker (2018), who use a difference-in-differences approach for disentangling the total treatment effect, providing an empirical application based on the Vietnam draft lottery. Secondly, the proposed estimation procedure is easy to implement, basing on a local regression to get the effect of the treatment on the outcome weighted by the treatment propensity scores that are straightforward to implement by a probit (or logit) estimation to get the potential values of the mediator. The estimation is compute in a bandwidth defined by Z.