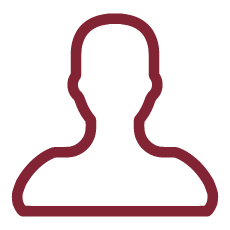
Climate change and migration are the current trend topics in developing studies. However, there is still a lack of consensus in the specialised literature on many important issues related to the causal relationship between increasing temperature, weather anomalies and migration decisions. This is becoming more and more central in the economic literature, with critical and far-reaching policy implications. In this context, our research project consists of four main goals: i) first to identify and remove common misconceptions in the analysis on weather shocks and climate change and their impact in triggering migration phenomena; iii) to conceptualize the role of migration as an adaptive strategy in managing ex-ante climate risk to foster current vulnerability and resilience assessments; ii) to innovate the empirical analysis of the role of climate change as a push factor for migration by using the most recent econometric techniques and new machine learning algorithms; iv) to make better use of micro data from international organizations to study long-run dynamics and draw key prescriptions to inform the policymaking process.
This project would bring relevant contributions to the literature under several different aspects. First, apart from rare exceptions, such as Cattaneo and Peri (2016), who make use of an econometric technique called ¿long differences¿ to explore migration responses to long-run climatic changes in several countries, our work would emphasize the need to bridge the external validity gap that so often affects the studies on the climate-migration relationship and, more generally, the entire new weather-economy literature. These external validity issues stem from the fact that, despite many works promise to explore the migration response to long-run climate changes, what they often carry out is just an empirical analysis based on short-run dynamics, i.e. migration decisions in response to annual shocks or temporary droughts. This is not equivalent to studying the impact of climate change on migration. The extrapolation from short-run elasticities to long-run trends determines a gap in terms of external validity, making these studies vulnerable to concerns on whether short-run relationships would also hold in the long-run, as both intensification of climatic effects and extensive adaption will take place (Dell, Jones & Olken, 2014). Our proposal is based on the use of: i) long time differences, i.e. econometric specifications using decadal climatic averages in place of annual weather shocks as covariates of interest, on the wake of Cattaneo and Peri (2016); ii) a simultaneous estimation of weather shocks and climatic trends on migration responses, to disentangle the effects of short-run anomalies from those of long-run, permanent changes in climate. This is an improvement over previous literature, as it would produce more reliable and robust results for policymakers. iii) the identification of evidence-based resilience thresholds to weather anomalies. This can be done by taking advantage of both the FAO Resilience Capacity Index (RCI) and the World Bank LSMS-ISA panel data.
Second, the use of recently developed machine learning algorithms would represent a major added value to study migration phenomena. Machine learning is causing a revolution in many science domains, and economics is no exception. Machine learning complements traditional econometrics by providing a set of algorithms (both supervised and unsupervised) that have been proven to be far superior than traditional methods when it comes to prediction. Prediction and causality are radically different purposes: when the aim is to predict an outcome as accurately as possible, the confidence intervals and reliability of the estimated parameters of the covariates become relatively less important. In this project we would explore both prediction and causality.
Another advantage of machine learning is that the distinction between cross-sections and panels, so critical in economic research, is less important when using machine learning. This is a huge advantage for this project as we plan to work with micro data from developing countries, where long panels are often unavailable even when using the gold-standard micro data such as the above mentioned LSMS-ISA or the Demographic and Health Surveys by the World Bank. This relative independence of machine learning techniques from panel data will allow us to formulate predictions even in absence of panel data and simply relying on repeated cross sections.
Finally, we would share new light on the timing and mechanisms of the migration decisions. The standard literature looks at migration as a post-shock coping strategy implemented by poorest households or individuals that are hit hard by the shock. The most recent perspective looks at migration as a collective decision acting as an ex ante insurance strategy. It helps to manage risk, natural and man-made, reinforcing resilience and vulnerability. As such, only better-off households will be able to send members elsewhere and thus rely on them to smooth the negative effects of climate shocks and cope with reductions in income or consumption. In this sense, migration is also a resource and an investment for richer households. This is particularly the case when we talk about climate-induced migration: climate change is a negative shock that will take place gradually and only hit in the long-run. It is thus a context in which it is reasonable to suppose that rational households will implement risk management strategies to cope with their expected vulnerability and that migration decisions will play a key role in household adaptation strategies. Our project would bring new empirical evidence on these relatively unexplored causal links, thus bringing together the migration literature with the development literature that focuses on risk and vulnerability.