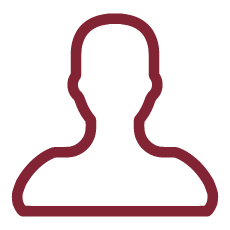
Management of cancer patients is challenging. At present, multidisciplinary unit, involving different professional figures from all relevant specialties, is an essential entity to assure the highest cure rate, independently of primary tumor site. A rigorous pre- and post-treatment multidisciplinary work-up is required to plan the more appropriate therapy and follow-up program.
Despite significant progress in the conventional modalities of surgery, radiotherapy and chemotherapy in the vast majority of common - breast, prostate, lung, colorectal - and uncommon - anal canal, head and neck - cancers, conclusive evidence of novel strong and independent prognostic factors for survival in cancer patients is lacking. Due to advance knowledge of the genetic mechanisms, biomarkers for cancer activity and optimal treatments, other favorable prognostic factors associated with tumor could be ruled out as an explanation for survival difference. To promote and increase patient stratification, a machine learning approach should be proposed and each multidisciplinary unit should serve as a platform to accumulate clinical data. The hope is to define robust tools to improve decision-making process.
This study will use machine learning methods to analyze follow-up data in oropharyngeal patients treated with curative IMRT and concomitant chemotherapy. In the published oropharyngeal cancer literature, the amount of direct evidence on this issue is scarce. To date, prior analysis attempted to categorize patients into low-, intermediate- and high-risk groups [1]. Survival oropharyngeal data from the Radiation Therapy Oncology Group (RTOG) 0129 trial were retrospectively examined to generate a predictive model [1-2]. In total, 266 patients with oropharyngeal squamous cell carcinoma were included in a recursive-partitioning analysis. HPV status was the major OS determinant. In addition, tobacco smoking and N stage for HPV-positive cancers or T stage for HPV-negative disease were proposed as independent factors with influential predictive significance [1]. Patients were then classified into low-, intermediate- or high-risk groups, with respect to the risk of death (7%, 30% and 54%, respectively). To our knowledge, our analysis will be the first study that will investigate the potential decision tree application in oropharyngeal cancer patients in a modern context, including 8th TNM staging system and IMRT technique. We will introduce for the first time the decision tree approach in oropharyngeal cancer data analysis. The predictive information should provide an effective and accurate decision making in the management of patients with oropharyngeal cancer. In fact, we hope to propose a classification tree that could help to guide future research in oropharyngeal cancer field. A decision tree approach will show OS probability for the groups in which our entire sample will be partitioned. The partition will be dependent on the important variables more commonly selected in randomForest to provide a robust assessment of the predictors having the best explanatory power in the problem at hand. Identifying oropharyngeal cancer risk groups could have important implications for treatment strategies, as well as follow-up program, in order to explore different schedules to improve outcomes. The final partition would be appropriate and clinically applicable.
References
[1] Ang KK, Harris J, Wheeler R, Weber R, Rosenthal DI, Nguyen-Tân PF, Westra WH, Chung CH, Jordan RC, Lu C, Kim H, Axelrod R, Silverman CC, Redmond KP, Gillison ML. Human papillomavirus and survival of patients with oropharyngeal cancer. N Engl J Med. 2010;363(1):24-35.
[2] Nguyen-Tan PF, Zhang Q, Ang KK, Weber RS, Rosenthal DI, Soulieres D, Kim H, Silverman C, Raben A, Galloway TJ, Fortin A, Gore E, Westra WH, Chung CH, Jordan RC, Gillison ML, List M, Le QT. Randomized phase III trial to test accelerated versus standard fractionation in combination with concurrent cisplatin for head and neck carcinomas in the Radiation Therapy Oncology Group 0129 trial: long-term report of efficacy and toxicity. J Clin Oncol. 2014;32(34):3858-66.