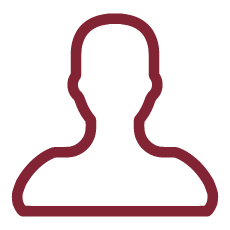
Observed social and economic phenomena are increasingly complex, both from a conceptual and an empirical point of view, as new technologies may be used to gather a huge amount of information. The available data are relatively new to statistical analysis, as only during the last decade we have enough computational resources to start dealing with them. Complexity arises either when the dimension of the observed data is large or when peculiar data are analyzed. One of the main tasks for modern statistical approaches is to develop new methods to cluster complex data structures. Clustering methods can either be model or heuristics based. In the first case, the framework is based on assuming that data are generated from a well-specified probabilistic model. Finite Mixture models are a powerful tool to represent a clustering structure in the data, where data arise from groups (also referred to as components) described by homogeneous density functions with cluster-specific parameters. The number of mixture components is an unknown parameter and several criteria have been proposed for its choice. In a Bayesian framework to parameter estimation, it can be hard to select the number of components as the corresponding posterior may be (and often is) flat. To avoid overly subjective priors, a solution maybe to consider infinite mixture models. Heuristic clustering methods are not based on a proper probability distribution for the observed data; rather, a (penalized) objective function to be minimized is often introduced. Standard clustering approaches should be modified to be applied to complex data, due to the high computational cost, and data complexity. Our project aims at defining clustering methods for such complex, high-dimensional, data; specifically, we will introduce novel data analysis methods and estimation algorithms. The latter will be included in software macros/libraries to be shared with the aim at helping practitioners working in the field.
Classical clustering approaches cannot be directly applied to complex data, due to both computational cost, and data complexity. As standard clustering methods fail, new methodologies, specifically tailored to such complex data structures, need to be introduced. Our project aims at defining clustering methods for complex, high dimensional, data; the aim is two-fold. First, we aim at defining original model and heuristics based clustering methods for high dimensional data. Besides the theoretical contribution, and in order to allow practitioners to use such methodological proposals in a friendly manner in everyday analyses we aim at producing efficient, well detailed, software implementation (mainly for the R software platform) which may greatly help the spread of such theoretical contributions. The analytical and modeling tools developed are expected to be used not only in the specific application domains that have stimulated these ideas. Rather, we expect them to be borrowed to several further research fields, such as social, economic, behavioral, psychometric domains, where data analyses and results can drive policy making and improve complex decision making. Currently, everyday life is in fact characterized by an impressive technological innovation process which has led to impressive big data storing. From this perspective, statistics is called to offer a fundamental support to meet the informative challenges raised by social needs. From a scientific point of view, we will pay great attention in this project activities to the (as wide as possible) dissemination of the results emerging from the research lines that have been previously described. The project will promote the production of research papers from doctoral, postdoctoral fellows and team members; these will be sent for publication in international journals, and presented at international conferences. The programming code developed, mainly in the R environment, to pursue parameter estimation fror the proposed methodological innovations will be made publicly available through either the CRAN R website or an appropriately designed page hosted by the department website. Such an approach has been proven to help make the methodological tools as accessible as possible to all potentially interested users and practitioners in a wide range of potential fields of research. A final scientific meeting on the themes of the project, with a view towards contributions to clustering high dimensional complex data will be organized, with the participation of team members as well as of national and international experts.
The proposed methodological advances will be applied to data coming from education, health, and social sciences. Besides these domains of application, all those empirical contexts which may be characterized by complex and high dimensional information on large samples could evidently benefit from new proposals and new solutions to these problems.
Furthermore, the methodological developments produced by the current project can have a strong impact on the Horizon 2020 challenge on "Health, demographic change and wellbeing" given the applied statistical skills of the research group as well as on other challenges due to the flexibility and use of clustering methods in several, different, applied domains.
To be more specific, our proposal is defined also to be coherent with, at least, two pillars of the Horizon 2020 framework program. In fact, the research project i) is aimed to produce "excellent science" by creating, in the long term, a scientific network between team members and other units collaborating from Italy and abroad, producing a stimulating environment for rich theoretical and empirical contributions. Further, due to high computational cost of the tackled statistical methods, an innovative aspect will be to handle it by defining a proper computing environment based on parallelization, by means of the department cluster;
and
(ii) it tackles some of the "societal challenges" described in the REGULATION (EU) No 1291/2013, "With the aim of deepening the relationship between science and society and reinforcing public confidence in science, (...) by making scientific knowledge more accessible, by developing responsible research and innovation agendas that meet citizens' and civil society's concerns and expectations and by facilitating their participation in Horizon 2020 activities".