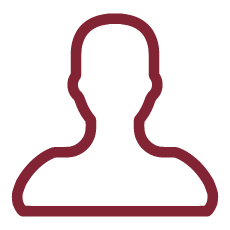
In the last years, we have experienced a substantial increase of applications in several empirical domains where high-dimensional data were entailed. With high-dimensional data we mean hundreds or thousands of variables for each unit in the observed sample. This has attracted the interest of a growing number of researchers due to the need of data reduction techniques in such applications, and several new approaches have been investigated is these areas.
Biclustering techniques have been proposed in several scientific fields especially to analyze data matrices where the two modes, which are usually units (rows) and variables (columns), can play the same role. In such cases, subsets of units may in fact be homogeneous only under a limited set of conditions (variables) while showing little similarities outside these.
One of the main tasks for modern statistical approaches to biclustering is to develop techniques for handling categorical (nominal and ordinal) and mixed-type data. Such data are encountered very frequently in practice whenever, for example, attitudes, abilities, or opinions are quantities of interest. However, practitioners often apply in such a context techniques developed for continuous data that can often be found to be inappropriate. This can lead to wrong results and, therefore, it would be worth taking the essential characteristics and features of these data into proper account to develop more appropriate techniques.
Our research project aims at defining new biclustering approaches for categorical and mixed-type data; specifically, we will start by extending clustering methods for categorical or mixed-type data in a two-mode setting, through both heuristic and model-based approaches. We will also look at extensions of such techniques to evaluate the impact of time in the model specification when longitudinal data (the units are followed in time) are available.
In behavioural, social and health sciences, the variables used to measure attitudes, abilities or opinions are typically categorical, frequently on an ordinal scale. In such contexts, different sources of heterogeneity may arise. In the last decades, an increasing interest in clustering categorical and ordinal data has been observed, but little work has been done if we compare the amount of proposals to the field of continuous data. In fact, modelling ordinal variables is quite challenging, due to the lack of metric properties; therefore, it is still common to ignore their nature by treating the ranks as interval-scaled. This can lead to wrong results; and, for these reasons, it is worth proposing techniques that take their characteristics and properties into proper account.
In some cases, the interest is to provide a joint clustering of units and variables that is, to partition the data matrix into homogeneous blocks with respect to some observed features. The task, which may be thought of as an extension of standard clustering approaches to group both units (rows) and variables (columns) of a data matrix, is often referred to as biclustering. In the case of ordinal data, such approach may be useful in several research fields, as for instance in marketing studies where finding a subset of customers that tends to evaluate similarly a subset of products or services may help to target some products or services according to customer profiles.
Our project aims at defining biclustering methods for categorical and mixed-type data; the aim is two-fold. First, we aim at defining original model and heuristics based biclustering methods for categorical and ordinal data, considering also a dynamic prospective. Moreover, to allow users to use the developed methodological proposals in a friendly way, we aim at producing efficient and structured software implementations (mainly in the R environment) which may greatly expand the spread of such methods. The developed software will be made available either through the CRAN (comprehensive R archive network) or a dedicated website. The analytical and modelling tools we aim to develop are expected to be used not only in a specific application field, but to be accessible to all potentially interested users and practitioners in a wide range of research fields such as social, health, economic, behavioural, psychometric domains, where data analyses can drive and improve policy and decision making.
From a scientific point of view, we will look at the diffusion of the results emerging from the research lines that have been previously described. The project will encourage team members to produce publications on international journals, and to participate at international conferences.
A final scientific meeting on the project themes, with a view towards contributions to biclustering categorical and mixed-type data will be organized, with the participation of team members as well as of international experts.
The methodological developments produced by the current project can have a strong impact on the Horizon Europe challenges due to the flexibility and use of clustering/biclustering methods in several, different, applied domains. Just to give an example, our proposal is consistent with the pillar of the Horizon Europe aiming at producing "Excellent science" by reinforcing scientific collaboration between team members and other cooperating units from Italy and abroad, producing a stimulating environment for rich theoretical and empirical innovations, especially for the participating PhD students. One of the current goals is to inspect the socio-economic consequences of COVID-19 in Europe: "The SHARE Wave 8 COVID-19 data allow examining in-depth how the risk group of the older individuals is coping with the health-related and socioeconomic impact of COVID-19." These data may be of different nature: developments of new modern statistical methodologies for analysing and summarizing mixed-type data in an appropriate manner may help to better characterize and interpret their genesis and evolution.