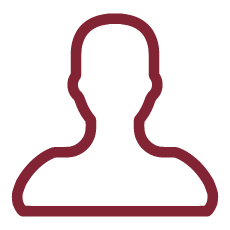
Natural Language Processing has long sought ways to reliably map a word or longer expression in context to some representation of its meaning. The most popular framing of this problem is so-called Word Sense Disambiguation (WSD), in which an algorithm has to classify the contextual target into a set of sense classes defined by a dictionary. However, WSD suffers from many intrinsic problems: excessively fine granularity of the inventories, extreme class imbalance, paucity of training data. In our research we tackle these issues by incorporating pre-existing knowledge into supervised WSD systems: glosses, graph relations, multimodal information. Moreover, we investigate alternative framings for computational lexical semantics, i.e. generating a gloss directly -- doing away with sense inventories completely; or embedding sense representation into graph-like representations of larger units like sentences.
Our work integrates, develops and adapts cutting-edge deep learning techniques for our research purposes. Being able to publish at the top tier conferences in the field means being judged able to produce a significant advance in the state of the art. Our work has already significantly outperformed alternatives in both Word Sense Disambiguation by proposing a stronger former of hybridization between supervised and knowledge-based algorithms (Bevilacqua and Navigli 2020) and in Semantic Parsing, by reducing the parsing task to sequence-to-sequence transduction (Bevilacqua et al. 2021). We have also opened up new research directions completely by presenting, for the first time, a sequence-to-sequence system being able define (at least in theory) any target-in-context (Bevilacqua et al., 2020). In the future we plan to further the state of the art by integrating novel powerful techniques, such as neural end-to-end retrieval (Lewis et al., 2020).
Bevilacqua M., Navigli R. - Breaking Through the (80%) Glass Ceiling: Raising the State of the Art in Word Sense Disambiguation by Incorporating Knowledge Graph Information, Proceedings of ACL (long paper), 2020.
Bevilacqua M., Maru M., Navigli R. - Generationary or: "How We Went beyond Sense Inventories and Learned to Gloss", Proceedings of EMNLP, 2020.
Bevilacqua M., Blloshmi M., Navigli R. - One SPRING to Rule Them Both:Symmetric AMR Semantic Parsing and Generation without a Complex Pipeline, Proceedings of AAAI, 2021.
Lewis P., Perez E., Piktus A., Petroni F., Karpukhin V., Goyal N., Küttler H., Lewis M., Yih W., Rocktäschel T., Riedel S., Kiela D. - Retrieval-Augmented Generation for Knowledge-Intensive NLP Tasks, Proceedings of NeurIPS, 2020.