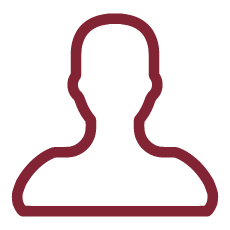
Prostate Cancer (PCa) is the second most common cancer in men worldwide and it is universally acknowledged as a complex disease, with a multi-factorial etiology. For long time, the major issues of prostate cancer management heve been rapresented by the non-targeted approach that have caused a high number of overdiagnosis and overtreatment, with the detection and treatment of clinically insignificant PCa (ciPCa), increasing overall costs and complications' rate.
The pathway of PCa diagnosis has changed dramatically in the last few years, with the multiparametric Magnetic Resonance (mpMRI) and the MR-directed targeted biopsies, playing a starring role and constituting the recently introduced "MRI Pathway" , that allowed to lower the rate of ciPCa.
In this scenario the basic tenet of Computational Medicine (CM) that sees the disease as perturbation of a network of interconnected molecules and pathways, seems to fit perfectly with the challenges that PCa early detection must face to advance towards a more reliable technique. Integration of tests on body fluids, tissue samples, grading/staging classification, physiological parameters, MR multiparametric imaging, Artificial Intelligence and molecular profiling technologies must be integrated in a broader vision of "disease" and its complexity with a focus on early signs. PCa research can greatly benefit from CM vision since it provides a sound interpretation of data and a common language, facilitating exchange of ideas between clinicians and data analysts for exploring new research pathways in a rational, highly reliable, and reproducible way. Thanks to the possibility given by this comprehensive approach, the definitive end-point is to avoid useless diagnostic procedure and disease overtreatment.
Every single tool described above has been singularly validated and some of them, such as MRI, represent the state of the art in PCa management. Up to date, CM has proved to be able to integrate a large number of genetic and -omics data for several different cancers including breast cancer, lung cancer and prostate cancer by combining for example reverse-engineered gene regulatory network with expression profiles computing the likelihood that genes and associated pathways are mediators of prostate cancer. Also, network-based approaches have been exploited to stratified cancer cohorts into clinically and biologically meaningful subtypes for different cancer types with marked heterogeneity, including ovarian, uterine, lung, and prostate cancer. For each tissue, by exploiting a network propagation algorithm and by clustering together patients with mutations in similar network regions, a robust patients division into molecular subtypes that were predictive of clinical outcomes, such as tumor histology, overall patient survival, and therapy response has been obtained.
The major innovations proposed by our approach is to integrate the above mentioned data altogether with clinical information derived from liquid biopsy on body fluids - on serum using Proclarix and on urine using SelectDX tests - and data obtained from MRI and ML application, by developing a computational algorithm that will provide a dedicated and user-friendly tool able to stratify risk according to patients' profiling with the ultimate goal of performing a minimally invasive and early diagnosis, that will lower the risk of initiating useless diagnostic and therapeutic pathways, that would increase overall costs and burden on the national health system, lower the number of overdiagnosis- and overtreatment-related complications, and allow the introduction of personalized therapy.
A future goal will be to develop and apply CM also for wide patient's secondary screening and for drug repurposing. In fact, CM methods could also aid drug discovery by exploiting shared similarities among drugs or diseases and infer similar therapeutic applications or drugs selection (drug repurposing)(18). The goal would be to build correlation networks composed of drug and disease perturbed genes, defined by genes that are upregulated (downregulated) by the disease and downregulated (upregulated) by the drugs. The greater the drug and disease genes sets correlation, the higher the likelihood that the drug is a viable candidate for repurposing. Patients stratification could be the most appropriate strategy to find highly efficient drugs for a given subgroup of patients that might have a lower effect in different subgroups.
To conclude, the basic tenet of CM, i.e., disease as a perturbation of a network of interconnected molecules and pathways, seems to fit perfectly with the challenges that PCa early detection must face to advance towards a more reliable and noninvasive technique. As a matter of fact, tests on body fluids, tissue samples, grading/staging classification, physiological parameters, multiparametric MRI, and many other molecular profiling technologies must be integrated in a broader vision of "disease" and its complexity, with a focus on early signs. In this regard, the field can benefit from the framework and algorithms of CM. Moreover, early detection is particularly important for PCa, since its early detection and treatment provide the greatest chance of cure. However, so far, performance relies upon human-based skills that vary considerably among dedicated health centers.
Finally, we envisage that PCa management in toto can greatly benefit from Computational Medicine vision and algorithms since it provides a sound biological interpretation of data and a common language (a metaphor) that facilitate the exchange of information between clinicians and data analysts for exploring new research pathways in a rational, highly reliable and reproducible way.
18.Turanli B, Zhang C, Kim W, Benfeitas R, Uhlen M, Arga KY, et al. Discovery of therapeutic agents for prostate cancer using genome-scale metabolic modeling and drug repositioning. EBioMedicine. 2019 Apr;42:386¿96.