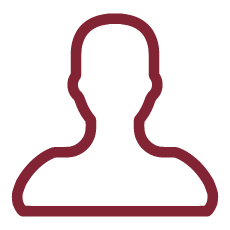
In Silico Clinical Trials (ISCT), i.e., clinical experimental campaigns carried out by means of computer simulations of computational models for human physiology, patho-physiology, Pharmacokinetics/Pharmacodynamics (PKPD) (aka Virtual Physiological Human, VPH, models), hold the promise to decrease time and cost for the safety and efficacy assessment of pharmacological treatments and biomedical devices, reduce the need for animal and human testing, and enable precision medicine, where personalised treatments optimised (or devices optimally configured) for selected individuals can be designed before being actually administered (or installed).
In this project we propose novel general-purpose computational methods and software (i.e., working on any VPH model) at the intersection of Artificial Intelligence (AI) and Model Checking to support uptake of ISCT in the early phases of the experimental assessments of new drugs or biomedical devices and model-based precision medicine.
We will evaluate our overall approach on two case studies in important areas of endocrinology: the female HPG axis and the glucose regulation mechanism in Type 1 Diabetes Mellitus (T1DM) patients. This is possible thanks to our multidisciplinary team and our external international partners.
[Max 5000 chars]
= Section 3 =
== 3.1. General methods for VP admissibility assessment ==
We will advance the state of the art ([22, 23]) as for VP admissibility constraint definition for VPH models whose parameters are not directly measurable and have unknown probability distributions. In particular, we will exploit and extend methods typical of model-based verification of Cyber-Physical Systems (CPSs) (see, e.g., [24] and references therein), by investigating the use of logics, automata and more general hybrid systems to capture and formalise domain (medical) expert knowledge in order to define admissibility constraints.
Such constraints will define necessary behaviours of a VP in different scenarios, i.e., under different medical actions and/or external events. We will also support the definition of inter-scenario constraints, i.e., constraints defining the consistency of a VP under multiple scenarios (e.g., the increase/decrease of glucose blood level in a T1DM patient under a higher/lower food intake and/or reactions to different insulin injections).
The goal is to reduce the problem of checking admissibility of a VP to a system-level verification problem (from the Model-Checking domain), where a VP plays the role of the system-under-verification, the possible scenarios play the role of the environment model and admissibility constraints play the role of safety/liveness properties.
== 3.2. General methods for generating complete cohorts of VPs ==
As outlined in Section 2.3, the problem of finding VPs for VPH models is central to enable ISCT. In particular, in our previous work [22, 23] we exploited statistical model-checking to generate cohorts of VPs. Those approaches however, although focussing on the same VPH model of our HPG axis case study, devise a model checking¿based algorithm specifically tuned against the model and propose admissibility constraints bounded to qualitative similarity of model trajectories. Also, they do not consider drugs PKPD.
In Pillar 1 we will advance the state of the art in generating cohorts of VPs by providing methods and software working on any (ODE-based) VPH model. This will be done by leveraging on the general admissibility constraint modelling methods mentioned earlier (which, differently from [22, 23], also take into account drugs PKPD and inter-scenario constraints) and on general-purpose massively-parallel intelligent search methods that guarantee completeness of the computed cohort (i.e., that it is large enough to adequately represent all relevant phenotypes), either with certainty or, if needed, with a given degree of statistical confidence.
Although ideally we also want the computed cohort to be minimal (i.e., not containing physiologically inadmissible phenotypes), achieving simultaneously both completeness and minimality is presently out of reach, because of the huge size of the parameter space of VPH models of practical relevance. Hence, by guaranteeing completeness (while also aiming for minimality, although with no guarantee), we place ourselves into a risk-conservative setting.
Stratification of the VPs in the computed cohort is also an important advancement, as it will allow ISCT to adaptively focus on specific phenotypes, also supporting prioritisation of patient sub-groups in follow-up in vivo clinical trials.
== 3.3. Classification of VPs according to measurable phenotype traits ==
Although several studies exist which characterise inter-individual variability within a population (see, e.g., [17], which combines Bayesian statistics with detailed mechanistic physiologically-based Physiologically Based PharmacoKinetic, PBPK, models), the problem of classifying a cohort of VPs according to measurable phenotype traits using clinical data is still open in the general case of VPH model (as ours) whose parameters are not directly measurable. This is the precisely the goal of Pillar 2.