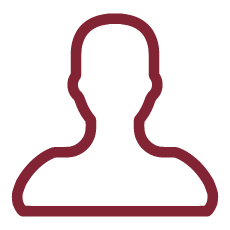
A covert channel is any communication channel that can be exploited by a process to transfer information in a manner that violates the systems security policy. In short, covert channels transfer information using non-standard methods against the system design. Covert communication channels techniques have been extensively studied and these channels are commonly intended to be used to protect privacy or to increase the security in critical communication scenarios. Nowadays covert channels and the way to actually implement them are covered by the field of steganography. But as every security concept, these techniques can be used in a malicious way, representing a new frontier for cyber-crime and cyber-espionage. Research is constantly working on finding new ways to covertly transmit information for the benign use of covert channels, and likewise malicious entities are looking to do the same but for a malicious purpose.
In this project we aim at showing that a recent proposed technique to train a machine learning (ML) model in a decentralized way (federated learning by Google), that includes thousands of participants can be used as a novel covert communication channel among participants of the learning scheme. We aim at characterizing this type of covert channel, in terms of the opportunities it presents but also the threats that it might impose in an infantile domain such as that of federated machine learning.
Actually there is no current technique that aim at communicating in a covert manner using as the medium of transmission the federated learning scheme. Mostly research has stated that the benefits of federated learning are very big when it comes to preserving the data privacy and also speeding up the training time and performance of the trained machine learning model, and international companies of the Information Technology sector have also put this research outcome into the actual products. For example, Google is actively using federated learning in order to improve the predictive models that they use in the Google Gboard application, which is the most common typing application found on all Android devices, being that Android operating system is maintained by Google.
In our research we want to show that federated learning can be used as a general purpose covert communication channel, but also while doing so we want to highlight and prove our belief that federated learning comes with some weak points that can be exploited by malicious adversaries to spread in a short amount of type, malicious payloads (such as ransomware, or keyloggers) and potentially cause a lot of problems.
Due to the benefits that have come with the use of federated learning (such as reducing the time to train a high quality model, protecting the privacy of the local data each participant has etc.) the research community has not focused particularly in understanding this paradigm well and find out its theoretical flaws and try to fix them. This is what we want to demonstrate and possibly fix in the course of this research project.