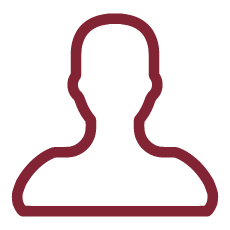
Nowadays, one of the main issues in statistics consists of the management of data coming from several different sources. Indeed, during the last years Statistics Institutes and academia have been engaged in the development of data integration techniques that would have allowed merging procedures. The availability of joint information is not only important per se, i.e. to have longer datasets, but it represents an actual need in many fields. Considering social sciences, one of the most interesting examples that clearly shows the cruciality of data integration is the problem of the estimation of the magnitude of the intergenerational mobility phenomenon when familiar data are not available.
The aim of my research is to implement and adapt new data integration techniques such as Record Linkage and Statistical Matching in social sciences' applications, exploiting both Bayesian and frequentist approaches. After a rigorous methodological comparison and the study of the properties of the proposed estimators, I will go further in the analysis relaxing some key assumption at the base of data integration methods that seem to be too binding in social sciences applications.
Implementing data integration methodologies in a socio-economic context would highlight new paths for interdisciplinary analyses, adding more scientific rigor to the matter.
Consider the aforementioned problem of intergenerational earnings elasticity measurement; at least two main issues arise. (i) Both variables of interest and collateral information are subject to measurement error; this leads to biased estimates; (ii) when the key variables are not very informative (or if they are not available at all), the econometric technique of Two Samples Two Stages Least Squares leads to a biased predicted distribution of the variable of interest, and consequently to a biased IGE estimate. Data integration techniques such as Record Linkage and Statistical Matching would be able to solve both issues at one time. Firstly, merging techniques are intrinsically able to keep the original distribution of the data unchanged. Secondly, it would be possible to include a measurement error correction model in the matching/linkage procedure via MCMC methods, in order to smooth the biased. This way, merging procedures would be able to produce less biased and potentially consistent estimates.
The definition of a measurement error correction model within a linkage procedure would not be an end to this application only. Such a model should be applied in other contexts; e.g., as an extension to the new small area estimation model with linkage uncertainty (Briscolini, Di Consiglio, Liseo, Tancredi, Tuoto 2018).
Beyond the specific applications, I would like to contribute relaxing key assumptions at the base of data integration techniques (mainly of Statistical Matching). In particular, the overcoming of the i.i.d. assumption is a relevant issue; although D'Orazio et al. (2006) presents some suggestions, further research in order to model the dependence structure of the observations (that would be temporal, as well as spatial) is still needed.