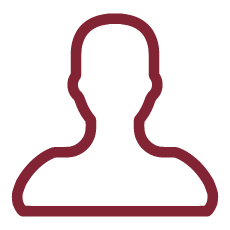
The need for integrating data and theory for optimal learning and forecasting strategies is now a widely recognized idea, rationalizing the initial overwhelming enthusiasm for exclusive data-driven models. The Bayesian perspective is particularly suitable for this type of approach, combining a priori hypothesis on the system with information extracted from data. We recently developed a framework based on the notion of the "adjacent possible," capable of explaining regularities observed in several systems related to human activities, at different scales, capturing both microscopic and macroscopic features. The underlying mathematical formalization of the proposed mechanism, leading to a Polya's urn like modeling scheme, turns out to be a generalization of a well-known (in mathematicians and computer science communities) stochastic process, namely the Pitman-Yor (or Poisson-Dirichlet) process, in turn, a generalization of the Dirichlet process. Those processes witnessed a renewed interest in the framework of Bayesian non-parametric inference for their suitability, when used in a hierarchical formulation, to uncover latent hierarchical structures, for instance in language modeling and clustering.
The urn models perspective opens the way to new improvements both in the theoretical understanding of this kind of processes and in their predictive abilities. This project aims to deepen this connection. On the one hand, we will investigate the limits of exchangeability to generalize inference techniques to the case of more realistic situations where temporal correlations are taken into account. On the other hand, we will study the hierarchical multi urns model, where the connections with the hierarchical processes discussed above are still not clear. In parallel, models on graphs (random walk on expanding graphs), that generalize the urn model to better account for complex interdependences and correlations in the system under study, will be developed.
This project opens completely new perspectives on stochastic processes widely used in Bayesian non-parametric inference. By elucidating the connection between the Bayesian framework, underlying stochastic processes and innovation dynamics modeling schemes (urn models and their counterpart as random walks on evolving graphs), it allows a deeper understanding of prediction strategies on systems featuring innovation. The project will mainly analyze databases related to human activities, but its finding could also be relevant in the analysis of biological systems so that to contribute to a predictive theory of evolution (Lässig et al., Nature Ecol. Evol. 2017). The possibility to overcome exchangeability, pointing to a more realistic time dependence of the posterior probability in a Bayesian framework, is a very relevant topic and could lead to important generalizations of existing inference schemes.
On a more theoretical ground, the present project proposes for the first time a complex systems view on Dirichlet-like processes. Those processes are related to the so-called "Sampling of Species Problem" or "Unseen Species Problem." Suppose we want to estimate the number of species belonging to an animal population. If one starts counting the different species upon encountering them, there will always be species never encountered. At each time step one can thus ask what is the probability to encounter a new species in the near future, or, better stated, whether the new encounter will be with an old (i.e., already seen) species or with a new one. Mathematically this corresponds to estimate the rate at which new events occur, and this is a very tough problem because it implies estimating the probability of events that never happened before. This is the general problem one faces when studying what is new: estimating its probability implies to be able to make accurate predictions about unseen events.
The typical problem of inference is that of estimating the probabilities of future events based on the observation of the past. When brand new events are possible, the inference scheme has to be revised. It is interesting to report a passage from a review by Zabell on this subject (Zabell, Synthese, 1992):
'This is not the problem of observing the "impossible", that is, an event whose possibility we have considered but whose probability we judge to be 0. Rather, the problem arises when we observe an event whose existence we did not even previously suspect; this is the so-called problem of "unanticipated knowledge".'
This problem is an old one. The present project precisely addresses this problem through a combination of a complex-systems with a machine learning perspective.
WP1: The project will parallel a thorough analysis of the role of different statistical measures in characterizing systems featuring innovation (and possibly identifying different "universality classes") with the design of modeling schemes able to account the observed universalities. The models in this area are still in their infancy, and we aim at providing the scientific community with new data-driven modeling schemes.
WP2: The project will deepen the connection between a complex-systems perspective and the Bayesian non-parametric framework, in particular building on recent advancements in the innovation dynamics field. By exploring the possibility of generalizing well-known stochastic processes through a more flexible reformulation in terms of urn models, we aim at providing the scientific community with new predictions schemes.