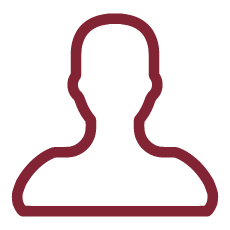
Some decomposition methods were proposed for variational inequalities (VIs) and Nash equilibrium problems (NEPs), but there is almost none about their more general and powerful versions: quasi-variational inequalities (QVIs) and generalized Nash equilibrium problems (GNEPs). For this reason we want to develop new decomposition algorithms for QVIs and GNEPs:
- We intend to generalize a recently proposed augmented Lagrangian method for QVIs and GNEPs in order to make it suitable for parallel computing. The applicability of this method is subject to monotonicity of the inner VIs/NEPs, and can be obtained for some classes of problems.
- We want to develop a fast decomposition method for QVIs and GNEPs that exploits a penalization approach to compute a solution by means of a sequence of gradient projection iterations. Specifically, relaxing the coupling constraints, each gradient projection iteration can be performed in parallel by resorting to a Jacobi-type decomposition. We want to define assumptions for stong monotonicity of the obtained gradient projection operators to show complexity measures.
- We want to better investigate the convergence properties of some recently proposed gradient-type projection method for QVIs in order to enlarge the class of problems solvable with a distributed procedure. We also intend to consider inertial terms to speed-up these methods.
- We intend to develop a new active set block coordinate descent algorithm to compute stationary solutions of some QVI equation system reformulations.
Our decomposition methods will significantly improve the literature on QVIs and GNEPs. From a theoretical viewpoint, we will widen the classes of problems solvable with gradient-like and distributed methods. And we will prove new conditions for the absence of non-optimal stationary points for QVI equation system reformulations. From a practical viewpoint, our distributed methods will be very efficient and can be used to tackle big data applications.
In the literature some decomposition methods were proposed for variational inequalities (VIs) and Nash equilibrium problems (NEPs), but there is almost none about their more general and powerful versions. This is exactly why we want to develop different decomposition methods for quasi-variational inequalities (QVIs) and generalized Nash equilibrium problems (GNEPs). The decomposition methods we want to propose, on the one hand, are very efficient because of the expected computational time-saving resulting from a distributed or parallel implementation, and, on the other hand, allow the distribution of the data in blocks that are assigned to separate processes, with a corresponding memory-saving that can be fundamental when dealing with big data applications.
We intend to generalize the augmented Lagrangian methods proposed in [1] and [2] for QVIs and GNEPs, respectively, in order to make them suitable for distributed/parallel computing (objectives (1) and (4)). This, beyond having practical advantages, is interesting from a theoretical point of view because completes the picture about augmented Lagrangian methods for variational problems.
We want to adapt the distributed paradigm proposed in [3] for optimization problems to both QVIs and GNEPs (objectives (2) and (5)). This point for us is of great theoretical interest. To develop these distributed algorithms we need to recall the well-known concept of approximate solution for GNEPs [4,5] and to introduce it for QVIs. The most relevant expected theoretical result consists of defining the firsts decomposition methods for QVIs and GNEPs with complexity bounds. That are algorithms with both a practical and a theoretical nice convengence speed.
Our study on the recently proposed gradient-type projection method for solving QVIs [6] (objective (3)) is theoretically interesting because: (i) it will enlarge the class of QVIs solvable with gradient-type projection methods and therefore with distributed methods, (ii) it will introduce in the QVI field the inertial speed-up term that seems to be very promising from a computational point of view.
As a first step to accomplish our objective (6), we want to define wide classes of QVIs that can be solved by computing stationary points of some optimization-problem/equation-system reformulations from the literature. Specifically, the optimality system of a QVI can be reformulated as a nonsmooth equation or a constrained equation with a smooth function [6,7]. Both reformulations can be exploited by algorithms and their convergence to solutions usually relies on the nonsingularity of the Jacobian or the fact that the merit function has no non-optimal stationary points. In this perspective, we want to prove new sufficient conditions for the absence of non-optimal constrained or unconstrained stationary points.
Finally using an active set block coordinate descent algorithm to compute the above mentioned stationary solutions would provide a very fast decomposition method for QVIs.
[1] Kanzow and Steck: Augmented Lagrangian and exact penalty methods for quasi-variational inequalities. Comput. Optim. Appl. 69, 2018
[2] Kanzow and Steck: Augmented Lagrangian methods for the solution of generalized Nash equilibrium problems. SIAM J. Optim. 26, 2016
[3] Colombo and Sagratella: Distributed algorithms for convex problems with linear coupling constraints. J. Global Optim. 2019
[4] Sagratella: Computing equilibria of Cournot oligopoly models with mixed-integer quantities. Math. Meth. Oper. Res. 86, 2017
[5] Sagratella: Algorithms for generalized potential games with mixed-integer variables. Comput. Optim. Appl. 68, 2017
[6] Dreves et al.: On the solution of the KKT conditions of generalized Nash equilibrium problems. SIAM J. Optim. 21, 2011
[7] Facchinei et al.: The semismooth Newton method for the solution of quasi-variational inequalities. Comput. Optim. Appl. 62, 2015