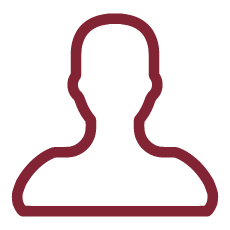
Ultrasonography is the primary tool for diagnosis and initial risk stratification of thyroid nodules. Some open issues limit its usefulness: 1. limited diagnostic accuracy of single sonographic features; 2. inter-observer variability; 3. inconsistent definition of critical features. Furthermore, when cytology is performed, in some cases, it renders indeterminate results. In these cases, sonographic risk stratification may also provide guidance.
Several approaches have been proposed to improve the diagnostic accuracy and the reproducibility of thyroid sonographic evaluation. It was reported that the use of computer-aided diagnosis systems to differentiate malignant from benign nodules showed accuracy similar to that obtained by radiologists and may reduce intra- and inter-observer variability.
This study aims to develop a new thyroid nodule ultrasound classification system based on a deep learning approach. The process will include generating a sonographic image database large enough (10'000 images) to contain examples of all predictive features and adequately train the system. This approach could also lead to the discovery of new US features that could be identified during the training or a better definition of the known ones. The system will be subsequently validated in an independent validation cohort and compared to current TIRADS (Thyroid Imaging Reporting and Data systems).
Several approaches have already been proposed to improve the diagnostic accuracy and the reproducibility of US evaluation of thyroid nodules, such as systematic reporting schemas (Leenhardt, et al. 2013; Russ, et al. 2017; Su, et al. 2014) and quantitative evaluation of echogenicity (Grani, et al. 2015). According to some evidence, thyroid computer-aided diagnosis (CAD) using artificial intelligence may further improve diagnosis reliability. It was reported that the use of thyroid CAD to differentiate malignant from benign nodules showed accuracy similar to that obtained by radiologists (Chang, et al. 2016; Choi, et al. 2017) and may reduce intra- and inter-observer variability.
Our aim is to develop a new thyroid nodule ultrasound classification system based on a deep learning approach. The process will include generating a thyroid nodule ultrasound image database large enough to contain all predictive features and adequately train the system. This system could also lead to the identification of new US features that could be identified during the training.
References
Chang Y, Paul AK, Kim N, et al. Computer-aided diagnosis for classifying benign versus malignant thyroid nodules based on ultrasound images: A comparison with radiologist-based assessments. Med Phys 2016; 43:554.
Cho J, Lee K, Shin E, Choy G & Do S. How much data is needed to train a medical image deep learning system to achieve necessary high accuracy?. arXiv:1511.06348, 2016.
Choi YJ, Baek JH, Park HS, et al. A Computer-Aided Diagnosis System Using Artificial Intelligence for the Diagnosis and Characterization of Thyroid Nodules on Ultrasound: Initial Clinical Assessment. Thyroid 2017; 27: 546-552.
Durante C, Grani G, Lamartina L, et al. The Diagnosis and Management of Thyroid Nodules A Review. Jama-Journal of the American Medical Association 2018; 319:914-924.
Erickson BJ, Korfiatis P, Kline TL, et al. Deep Learning in Radiology: Does One Size Fit All? J Am Coll Radiol 2018;15 (3 Pt B):521-526.
Figueroa RL, Zeng-Treitler Q, Kandula S & Ngo LH. Predicting sample size required for classification performance. BMC Medical Informatics and Decision Making 2012;12:8.
Gharib H, Papini E, Garber JR, et al. American Association of Clinical Endocrinologists, American College of Endocrinology, and Associazione Medici Endocrinologi medical guidelines for clinical practice for the diagnosis and management of thyroid nodules - 2016 update. Endocr Pract 2016; 22:622-639.
Grani G, D'Alessandri M, Carbotta G, et al. Grey-Scale Analysis Improves the Ultrasonographic Evaluation of Thyroid Nodules. Medicine 2015; 94(27):e1129.
Grani G, Lamartina L, Ascoli V, et al. Ultrasonography scoring systems can rule out malignancy in cytologically indeterminate thyroid nodules. Endocrine 2017;57:256-261.
Grani G, Lamartina L, Cantisani V, et al. Interobserver agreement of various thyroid imaging reporting and data systems. Endocrine Connections 2018;7:1-7.
Haugen BR, Alexander EK, Bible KC, et al. 2015 American Thyroid Association Management Guidelines for Adult Patients with Thyroid Nodules and Differentiated Thyroid Cancer: The American Thyroid Association Guidelines Task Force on Thyroid Nodules and Differentiated Thyroid Cancer. Thyroid 2016; 26:1-133.
Leenhardt L, Erdogan MF, Hegedus L, et al. 2013 European Thyroid Association Guidelines for Cervical Ultrasound Scan and Ultrasound-Guided Techniques in the Postoperative Management of Patients with Thyroid Cancer. European Thyroid Journal 2013;2:147-159.
Na DG, Baek JH, Sung JY, et al. Thyroid Imaging Reporting and Data System Risk Stratification of Thyroid Nodules: Categorization Based on Solidity and Echogenicity. Thyroid 2016; 26:562-572.
Rajpurkar P, Irvin J, Zhu K, et al. CheXNet: Radiologist-Level Pneumonia Detection on Chest X-Rays with Deep Learning. arXiv:1711.05225, 2017.
Russ G, Bonnema SJ, Erdogan MF, Durante C, Ngu R & Leenhardt L. European Thyroid Association Guidelines for Ultrasound Malignancy Risk Stratification of Thyroid Nodules in Adults: The EU-TIRADS. European Thyroid Journal. 2017;6(5):225-237.
Shen D, Wu G, Suk H. Deep Learning in Medical Image Analysis. Annu Rev Biomed Eng. 2017;19:221-248
Shin JH, Baek JH, Chung J, et al. Ultrasonography Diagnosis and Imaging-Based Management of Thyroid Nodules: Revised Korean Society of Thyroid Radiology Consensus Statement and Recommendations. Korean J Radiol 2016; 17:370-395.
Su HK, Dos Reis LL, Lupo MA, et al. Striving toward standardization of reporting of ultrasound features of thyroid nodules and lymph nodes: a multidisciplinary consensus statement. Thyroid 2014; 24:1341-1349.
Tessler FN, Middleton WD, Grant EG, et al. ACR Thyroid Imaging, Reporting and Data System (TI-RADS): White Paper of the ACR TI-RADS Committee. J Am Coll Radiol 2017;14:587-595.