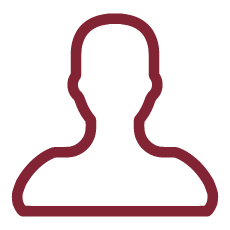
Recent advances in sensing technologies have enabled the generation of huge amount of spatio-temporal data, which represent a valuable asset for a wide range of application domains. Due to the sheer volume of spatio-temporal data that today companies need to process, in many application domains spatio-temporal data processing requires large computing power and, at the same time, real-time guarantees. As a results, a lot of applications are currently faced with a number of challenges that are not addressed satisfactorily by existing data processing platforms. This project aims at exploring the design of efficient tools for supporting real-time processing of spatio-temporal datasets in the cloud. In particular, the project will pursue the objective of minimize the operational and administration costs of the user cloud platforms via pervasive self-tuning mechanisms, able to build large computing platforms on-demand and to optimize them based on the different real-time processing constraints of computations. The final goal of the project is to develop effective tools that can open new opportunities in a number of application domains in which real-time processing of spatio-temporal data is increasingly required.
The goal of this project is to develop smart tools for automatizing and making cost-efficient the management of computing platforms for processing spatio-temporal data in the cloud. The objectives of these tools include: 1) identify the most cost-efficient type and amount of computing resources offered by cloud providers for the data processing tasks that the user needs to run, 2) building and resizing the computing platform on-the-fly to comply with the user task execution plan, 3) deploying the data processing algorithms on the machines to meet the processing deadlines.
The main contribution to the current state of the art of technologies for data processing in the cloud will be the introduction of new a holistic approach to self-tuning, aimed at pursuing optimal efficiency across the various levels of the platform. Previous research has revealed that a plethora of factors and parameters influence of big data analytics jobs. This makes defining a single model capable of capturing all such relations an extremely challenging task. To cope with this issue, we intend to investigate novel self-tuning schemes, based on a modular approach based on a divide and conquer approach and hybrid performance modeling. The key idea is to decompose the problem of identifying a globally optimum configuration for a set of components into the problem of coordinating a set of self-tuning mechanisms, each optimizing an individual part of the system. This approach will yield two key advantages: i) simplification of the global optimization process, compared to classic monolithic optimization approaches, by requiring to solve a set of simpler optimization problems; ii) support for the adoption of hybrid optimization techniques, i.e., employing different self-tuning methodologies (e.g., based on analytical modeling vs machine learning, on offline learning vs exploration) for different system's components.