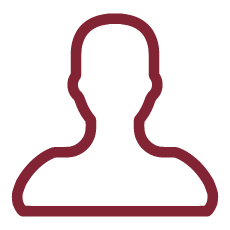
In the last decades, there is a growing interest in studying how data-driven decision-making models are impacting the real world. Even though it is straightforward to appreciate the advantages given by the usage of a data-driven model, there is a wide range of situations where algorithms are having controversial impacts. For instance, outcomes of models tend to favorite/discriminate one group over another, or in social network we observe phenomena like polarisation and, consequently, echo chamber formation. At the bottom of this, there is one issue and concerns about data we make a decision on top: it is generated by humans thus suffers from bias inherited from social structure.
On this perspective, the purpose of this research is to define cutting-edge methods that facilitate and generalize the detection and mitigation of bias. In particular, we want to provide the definition of a pre-processing technique that filters out hidden bias on a general dataset with respect to a class attribute and independently of the algorithm that is applied later on. Then, we focus on the detection of bias in a real case scenario: Wikipedia. Specifically, addressing this problem we design novel techniques to quantify the bias that a colored network structure implies to user navigation behavior.
Both directions might have a lot of impacts. The pre-processing technique represents a big step forward since respect to the context-dependent existing methods, it is general and applicable to any dataset. On the other hand, Wikipedia bias detection is a new problem, never addressed before, and if it turns out to be true there will be effective actions Wikipedia will have to take.
The project finds its roots in a field that is quickly growing and attracting attention from media, people, researchers, and governments. Its importance and centrality in current debates are demonstrated by the efforts of EU and US in controlling discriminatory and biased phenomena grasped by algorithms, through the writing of laws that act and pave the way for new algorithm implementations. On the other hand, a bunch of scientific communities, pushed by governments interest, are duplicating the number of publications in the field such that, new venues, focused on the topic, have been established.
To get an idea about the impact that research on this field might have, it is worth to mention cases where not controlled data-driven decision-making pipelines already causes unintended discrimination or polarization. In US, algorithms are used to predict recidivism, the likelihood of a person convicted of a crime to offend again. It has been shown that the recidivism for black people tends to be greater than that of white even if the phenomenon is not observed in reality [15]. In recent years the power of social network in politics debate is increasing a lot. Legal trials have been issued regarding the information manipulation and spreading of partial information that parties/candidates have coordinated for their escalation.
Working on mitigating such impacts is important for ethical reasons and citizens safeguard.
Algorithmic bias and fairness is still a quite new research topic and suffers from a lack of generalization. This condition opens two different paths: defining techniques that can enforce/ensure fairness regardless of the context they are applied, look for new use cases where bias has not been investigated yet and it might be a threat.
Our proposal, with respect to the reported state of the art, tries to go beyond limits current techniques suffer and proposes new approaches to deal with real scenarios that have not been explored yet.
The pre-preprocessing method we propose achieves two things the others do not: it can be applied to any dataset before feeding it to an algorithm and will be designed such that can be used for problems where sensitive attributes have more than two values. In this perspective, we are proposing a technique that generalizes the idea of getting rid of hidden bias from dataset at an early stage of the decision-making pipeline not involving anymore the pre-processing tuning/bias measurement required by other techniques.
Regarding Wikipedia bias detection - not in terms of content, rather in terms of network structure that induce some strange, biased and unfair user navigation patterns - this is a problem that has never been addressed before. We think that the outcome of this work might be very important for Wikipedia maintainers and Wikipedians. Indeed, due to the significant role that Wikipedia has, a source of knowledge on the Web, it can give insight and directions for the definition of a policy to manually and automatically monitor the phenomenon. If we prove the presence of bias and relative tools to mitigate its effects, it will have worldwide sanitization effects.
Technically speaking, to verify the presence of bias we will also propose a new technical approach, like property testing, that has not been used so far in polarisation/bias problem on networks and need to be customized accordingly.
[15] Fair prediction with disparate impact: A study of bias in recidivism prediction instruments. A. Chouldechova ,2016.