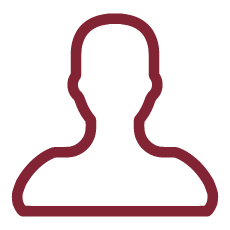
Urine cytology samples constitute a significant percentage of daily non-gynecologic cases in any cytopathology laboratory, and one of the most difficult specimens that pathologists encounter. The most relevant problems are the low sensitivity to detect low-grade, non-invasive lesions, absence of standardized diagnostic criteria, and interobserver variability. To meet this need, the Paris System 2016 classification proposed a standardized reporting system, including specific diagnostic categories and cytopathological criteria for the differential diagnosis of atypical cells. However, this method is based on a number of objective and subjective criteria (e.g., cell atypia, irregularity, and hyperchromasia), making detection an extremely time-consuming operation for clinicians. In this project, we plan to develop an automatic support method for detecting urothelial cancer cells from image data, based on recent progresses in the field of deep neural networks (DNNs). DNNs have become a de-facto standard in the computer vision field, and they have shown promising results in other medical scenarios, such as segmentation of chest radiographies. At the same time, out-of-the-box DNNs need large amounts of (expertly curated) data, and they tend to be poorly interpretable and overconfident in their predictions. Broadly, the aims of this project are (i) collecting a large database of urine cytology images classified according to the Paris System; (ii) implementing a state-of-the-art deep network to automatically classify, with high accuracy, new samples; (iii) carefully study the robustness, calibration, and interpretability of the network; and (iv) design a test scenario to gather data on the interaction between the trained system and real clinicians. The system offers the possibility of exploiting recent artificial intelligence techniques in urothelial cancer cell detection, significantly increasing sensitivity, accuracy and diagnostic reproducibility.
In the present project we can identify several innovative components that can greatly benefit research in cytology and deep learning support to healthcare.
One key aspect of deep learning is that it can benefit from large amount of training data. In our case, a prerequisite is to set up and standardize a large dataset of cytopathology images, to create a cancer images archive to be released publicly. Importantly, the criteria and expertise obtained during this phase can provide important guidance for the future. Users would have the opportunity to learn morphological aspects through the identification of the various cell types using pathology image platforms for visualization, morphometric analysis, annotation, and management while dividing images in different ¿study sets¿ for machine learning applications. We note that a number of support tools for image labelling have been developed in the last years (e.g., LabelImg), which could be exploited here.
Importantly, a large dataset of high-quality cytopathology images is not yet available, especially in uropathology. Public release of such a dataset could provide an enormous boost to research in the field, as is already happening in related topics such as eye diagnostics and chest radiology. Public datasets can be used for easy comparison of benchmarks, quick prototyping of solutions, or even for the organization of public competitions to increase the international visibility of the problem.
Finally, a calibrated, interpretable, automatic diagnostic tool can be of great help to pathologists, especially those who operate in centers with few experience or in underdeveloped countries. For example, artificial intelligence-based diagnostic algorithms applied to mammograms are assisting in the detection of breast cancer serving as a ¿second opinion¿ for radiologist. It would also open up to the possibility of expanding the dataset from our hospital through cooperation with other institutions.