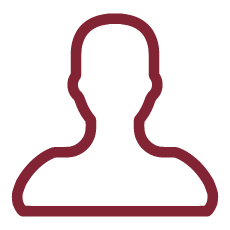
This research project focuses on the issue of automation, artificial intelligence (AI) and innovation-led growth. It builds on the recent theoretical and empirical literature on automation, robotics and AI that investigate the dynamic effects of introducing incentive to automation on R&D spending and economic growth. In this vein, the objectives of the research are the following:
1) to determine some stylized facts about the new frontier of digital technologies, robotics, automation and economic growth;
2) to construct a fully-fledged dynamic macro-model explaining data and stylized facts;
3) to use the derived theoretical framework to infer about the interactions between R&D investment, automation investment and growth;
4) to assess the effects in terms of workers attitude to work, international specialization, firm performance, and wage inequality;
5) derive policy guidelines for determining optimal R&D and automation spending.
This research wants to contribute to at least three research streams. First, the research is related to the literature on innovation and economic growth. Romer (1990) develops the seminal R&D-based growth model in which innovation is driven by the invention of new varieties of intermediates. Then, Segerstrom et al. (1990) and Aghion and Howitt (1992) develop the Schumpeterian quality-ladder model in which innovation is driven by the development of higher-quality products. Many subsequent studies use variants of the R&D-based growth model to explore the effects of R&D subsidies; see Peretto (1998), Segerstrom (2000), Chu and Cozzi (2018), Minniti et al. (2013) and Parello (2019). These studies do not feature automation, implying that capital intensity in the aggregate production function is exogenous or simply zero. This research wants to contribute to this literature by endogenizing the capital intensity of the economy and by focusing on automation and digital technologies as a driver for capital accumulation and economic growth
Second, this research is related to the literature on automation, AI and economic growth. Important contributions to this literature are the studies of Peretto and Seater (2013), Prettner and Strulik (2020), Chu et al. (2021a, 2021b). However, whereas Peretto and Seater (2013) and Prettner and Strulik (2020) propose, respectively, a quasi-AK model with ¿factor-eliminating¿ automation and a variety-expanding growth model with automation and endogenous education, our research will focus on a Quality-Ladder Schumpeterian growth model and will see automation as a cumulative input. In this respect, our research plugs into the rapidly growing literature on Schumpeterian growth and automation. In this vein, the studies closer to our research are Chu et al. (2021a, 2021b).
However, with respect to Chu et al. (2021a, 2021b), our contribution will consist in exploring different avenues through which automation can affect long-run growth and the design of R&D policy. In particular, in contrast with Chu et al. (2021a), who propose a quality-ladder model with hand-to-mouth workers and Zeira¿s (1998) machine-labor substitution process, our research aims at: 1) providing a different approach to model innovation and automation, where innovation will follow a stochastic process as in Minniti et al. (2013) and Parello (2019), and where automation can also be a by-product of the innovation activity; 2) eliminate the scale-effect from the steady state which causes the long-run equilibrium to turn explosive in the presence of a growing population; 3) revisit all of the policy results of Chu et al. (2021a), especially those concerning the existence of a trade-off between innovation and automation.
Third, this research is also related to the empirical literature that studies patterns of real income convergence employing the PS methodology; see Bartkowska and Riedl (2012), Lyncker and Thoennessn (2017), Cutrini (2019) for studies focusing on EU at a regional level, and Monfort et al. (2013), Borsi and Metiu (2015), Cavallaro and Villani (2020; 2021) for studies that focus on country-level analyses. These studies find no evidence for an overall cross-country economic convergence, but identify the formation of several convergence clubs. Differently from previous contributions, this research will focus on the convergence-divergence issue at the sector level and, upon identifying the clustered patterns of productivity growth, estimate the role of the specific innovation technologies adopted in the formation of clubs.
References (Selection)
Aghion P., Howitt P. (1992) A model of growth through creative destruction. Econometrica 60: 323-351
Bartkowska M., Riedl A. (2012) Regional convergence clubs in Europe: identification and conditioning factors. Econ Model 29: 22¿31
Borsi, M.T., Metiu, N. (2015) The evolution of economic convergence in the European Union. Empir Econ 48: 657¿681
Cavallaro E., Villani I. (2020) Real income convergence and the patterns of financial integration in the EU. North American Journal of Economics and Finance
Cavallaro E., Villani I. (2021). Club convergence in EU countries. A sectoral perspective. Journal of Economic Integration 36: 125-161
Cutrini E. (2019) Economic integration, structural change, and uneven development in the European Union, Struct Change Econ D 50: 102¿113
Lyncker K., Thoennessen R. (2017) Regional club convergence in the EU: evidence from a panel data analysis. Empir Econ 52: 525-553
Monfort M., Cuestas J.C., Ordonez J., (2013) Real convergence in Europe: A cluster analysis, Econ Model 33: 689-694
Peretto P. (1998) Technological change and population growth. Journal of Economic Growth 3. 283-311
Romer P. M (1990) Endogenous Technological Change. Journal of Political Economy, University of Chicago Press, 98 (5): 71-102
Segerstrom P. (2000) The long-run growth effects of R&D subsidies. Journal of Economic Growth 5: 277-305