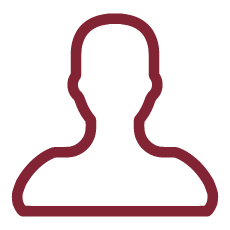
We live in a world where billions of smart objects, such as sensors and smartphones, collect huge amount of data and are connected together over an ubiquitous Internet of things (IoT) to offer better services in different application sectors. Mining information from massive volumes of data, and embedding intelligent networking of such heterogeneous devices in critical infrastructures such as the power grid, the Internet, or transportation networks, will revolutionize the way our systems are managed, enabling proactive sensing and optimization mechanisms aimed at ensuring guaranteed performance. There is no doubt that large economic growth, along with improvements in the quality of our lives, hinge upon an efficient management and design of IoT.
In this project, we plan to empower emerging IoT applications by providing fundamental advancement in the field of distributed online nonconvex optimization. We aim to develop a new class of online network algorithms promoting an adaptive and scalable design of the IoT system, while optimizing the user experience in heterogeneous, nonstationary, dynamic environments. Building on these fundamental tools, we plan to introduce strong advancements in two important IoT emerging tasks: fog-enhanced computation offloading, and distributed online big data analytics. In the former case, we aim at optimizing the joint radio and computational resource allocation of the fog network in order to minimize the average latency needed for offloading, while serving and making stable all the IoT workloads. Finally, in the latter case, our goal is to build a scalable IoT system enabling big data analytics via online deep learning, where fog nodes interact with each other and with the cloud in order to perform distributed training of deep architectures.
This project is highly innovative with respect to the current state of the art, and the results are expected to bring large innovation both from a theoretical and practical point of view.
In first place, we remark that the theoretical methods that the project will develop are completely novel, and do not represent a marginal increment of what is known in the literature. In fact, distributed optimization methods have been widely studied in the literature only in the static case, i.e., when objective functions of agents do not change over time, see, e.g., [21]-[36]. In the online setting, up to date, most of the efforts focused on the centralized convex case, see, e.g., [11]-[18]. On the other side, the online nonconvex case that we aim to pursue here is a largely uncharted territory, and there is plenty of room to produce fundamental contributions both in the centralized and distributed settings. In particular, while some centralized ONCO methods were already proposed in the literature, see, e.g., [19,20], to the best of our knowledge, this project is the first attempt to design fully distributed, theoretically provable, robust algorithms for online nonconvex optimization problems over large-scale dynamic networks. We will term this new field of research as DONCO, i.e., distributed online nonconvex optimization.
In second place, the developed algorithmic and theoretical results will serve as an exciting stepping stone to innovate online optimization tailored for contemporary IoT applications. In particular, existing approaches for fog-enhanced computation offloading in IoT either focus on static settings or assume stationarity of the environment [10], which might not hold in typical IoT applications involving, e.g., human interactions, or mobile networks. Furthermore, such methods are typically limited to convex IoT designs, see, e.g., [17,18]. Regarding deep learning (DL) methods, their merits are already well recognized in many practical applications such as speech and image recognition, natural language processing, computer vision, etc. [42,43]. However, DL implementations mainly focus on centralized algorithmic solutions, hinging on a large number of parallel processors working in a powerful cloud. Extending DL methods to latency-constrained distributed non-convex learning problems, such as those encountered when training deep architectures from massive data distributed over an IoT network, is still a challenging open problem, see, e.g., [44]. In summary, the exploitation of the proposed DONCO optimization methods in the IoT domain is expected to bring fundamental advances in terms of: (a) Adaptive, scalable, and flexible design and management of IoT; (b) Guaranteed user experience in heterogeneous, nonstationary, dynamic IoT environments; (c) Development of new distributed methods for fog-enhanced computation offloading in IoT networks; (d) Design of an innovative online big data analytics platform to extract information from huge amount of network data within latency constraints imposed by IoT applications; (e) A broader set of IoT tasks (not limited to fog computing and big data analytics) that can be formulated and solved within the proposed DONCO framework.
The theoretical objectives of this project are ambitious, since often they require the solution of challenging open problems. On the other side, the practical results achieved by the project will lead to significant advances in IoT design and management, which is expected to bring large economic growth and improvement in the quality of our lives. Finally, an important issue about this project, related to its fundamentally methodological aspect, is that the applicability of the proposed methods might have a very broad impact in different fields of knowledge or application, not necessarily related to IoT. Hence, it will be important, during the project to identify other fields from which this project can get useful sources of inspiration for further developments and towards which the methodologies developed in this project can provide useful tools. Some examples that come to mind are 5G networks, big data, Industry 4.0, but these are not the only possibilities.