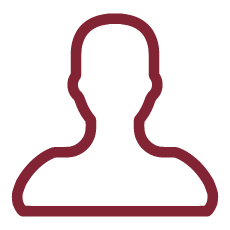
Preparedness and response of urban actors to different emergencies are among the main goals of several EU calls. A major issue occurring when rescuers leave for an emergency is the limited knowledge about the gravity of the problem. Also the intervention may not be immediate due to the physical distance of the rescue team from the emergency point or its commitment in a previous request. Providing situational awareness is widely recognized as an invaluable tool because it allows the rescue team to "see" what happens on the spot before intervening, properly planning the rescue mission.
This project aims at designing an autonomous and multipurpose squad of Unmanned Aerial Vehicles (UAVs), or drones, that can explore the area before the rescuers intervention and provide a view of the situation. The squad can be deployed on demand, offering a variety of first-response services (e.g., locating people who need help, making them communicate with the depot, picking up and delivering necessities). This is a challenging issue due to the potential vastness of the area: it is not possible to remotely control drones that are often disconnected from the depot.
Our project aims to be of aid to the human in the control loop, by making drone squads autonomous, self-configuring, and able to predict the type of required intervention. Our project will develop intelligent algorithms and predictive models that allow the UAVs squad to coordinate and take decisions on mission and trajectory planning, data routing, item delivery, and rescue prediction in a secure way. The research involved in this project is highly interdisciplinary, as it includes networking and management, machine learning, and blockchain aspects. We expect to revolutionize rescue operations supporting rescuers with a pre-mission view, to offer remote assistance (before being able to physically intervene) and plan an appropriate intervention. We believe this system will help save more lives.
From an application point of view this project will revolutionize the first response of urban actors to emergencies supporting public rescue and police authorities operations with a system that enables applications which were not previously possible. Specifically, we propose an efficient, secure, and reliable deployment of a squad of drones able to provide a pre-mission view to emergency authorities.
When a rescue team receives a request for intervention, the information regarding the seriousness of the incident is often unknown. Then the team may act in two ways: 1) a first team goes ahead to find out what happened and then calls additional help if needed (entailing a potential long delay in the intervention); 2) the team intervenes with all the available equipment, engaging devices and people that may not be needed. In both scenarios the team may not be able to intervene promptly because it may be physically distant from the emergency place, or because it may be busy in a previous commitment.
Providing situational awareness is widely recognized as an invaluable tool by rescuers because it allows them to ¿see¿ what happens on the spot before intervening, planning the rescue mission in the most appropriate way. A prompt and appropriate intervention allows to optimize the use of rescuers and equipment and hence to save more lives.
From a technical point of view the research proposed in this project is innovative in many ways.
Concerning the networking aspect, inter-adaptability between the network and the application is a totally new concept in squads of UAvs. We define inter-adaptability as the extent of match between application¿ requirements and network capacity in terms of currently available throughput. Most of current work addresses trajectory assignment and data routing, independently of the application needs. In this project we aim at performing routing by considering the applications needs. This means that if a drone is streaming data through a multi-path route, it can decrease video quality depending on the current communication network: intermediate nodes may have to relay traffic for multiple sources. Instead of decreasing the streaming speed, the source node could decrease the quality of its video so as to have a timely delivery that may contain crucial information even if with a lower quality.
Regarding mission planning and coordination, the innovative contribution stands for its capability of planning the whole mission, selecting and scheduling squads of drones that should execute multiple tasks, adapting the mission to changing needs at runtime, in combination with the adaptation to uncertain environment conditions and component failures. In this project we propose to provide models and algorithms to address the selection and planning problem and to address the runtime adaptation problem, that is, to analyze the system state and to plan the adaptation actions.
Federated learning seems a natural fit for UAV-enabled networks, although its application to real-world scenarios is still in its infancy. The main novel contribution of this work, therefore, is to demonstrate the benefits of FL when this is used to collaboratively train machine learning (ML) models on data collected by UAV devices for supporting rescue operations. Those models would support decision-making processes, especially in a time-critical state of emergency (e.g., determining the type/severity of an emergency by analyzing real-time image and/or video data gathered from the surrounding environment).
It is worth noticing that training such predictive models with the traditional centralized ML paradigm may not be a viable option for UAV devices, as they would need extensive raw data transfer from drones to the central server. Indeed, FL would greatly improve the network overhead and consume less bandwidth, which is pivotal for battery-constrained UAV devices. Moreover, FL would natively preserve the privacy of each UAVs local data, since those never leave the device where they are collected. Finally, FL would be effective for ultra low latency applications, as it does not require querying any remote, cloud-hosted predictive model at inference time.
Though promising, the adoption of blockchain systems as an infrastructure for UAVs communication is at its initial stages. In our project, we will tackle a number of still open challenges in the field. We will look into solutions that mitigate the usage of the available limited resources of UAVs, as several blockchain technologies are computationally greedy. Furthermore, we will pioneer the combined analysis of data stemming from the blockchain and UAVs to analyze the performance and execution patterns of the DaaS processes. We remark that the two information sources produce data that differ in granularity, structure and frequency of updates. Particularly in the context of this project, the joint examination of on-chain data and real-world events is a novel research avenue.