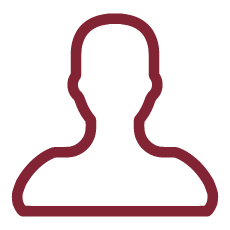
This research project aims at evaluating how Italian manufacturing firms reacted to the first Covid-19 outbreak. Emphasis is put on how firms' characteristics and behaviour affect their future performance.
A sample of firms has been interviewed right before and after the covid outbreak to investigate these features. The pre-covid survey covers a wide range of firms' characteristics, including financial and strategic components. The second survey has been conducted to capture the immediate effect of the shock on firms' organization and the change in their future expectations.
The evaluation will be conducted in the methodological frameworks of causal graphs and potential outcomes. The former will be used to recover the causal relations among the variables of interest and encode them into a graph. The obtained graph will then provide the necessary information to choose an identification strategy and estimate causal effects through potential outcomes methods. A novel methodology will also be proposed to deal with uncertainty in the graph structure when estimating causal effects.
The project investigates how firms' characteristics and reaction strategies to the covid shock affected their future performance. The behaviour of firms during the pandemic has been a central topic in research agendas in the last year. However, this proposal makes two important contributions to the existing literature.
The first element of innovation is related to the nature of the data. The dataset provided by MET captures a rich set of firm dimensions, including implemented strategies, workforce characteristics, value chain positioning and financial structure. Having such a rich dataset is an added value compared to similar studies and is a key element to obtain a comprehensive representation of the problem and make causal estimates more credible. Moreover, to the author's knowledge, none of the recent works on the topic evaluates the impact of a firm's behaviour after the shock on their future expected performance from a causal perspective.
The second contribution is methodological. For years potential outcomes have been the reference framework when dealing with causality in economics. On the other hand, causal graphs have met success in other disciplines where causal queries usually involve a high number of variables, such as epidemiology and biostatistics. Implementing causal graphs in this kind of economic analysis would mean being able to deal with more complex causal models and exploiting its causal discovery features. In fact, when causal relations between variables are not known a priori, these can be extracted from a dataset through structural learning algorithms. The causal structure is then encoded into a graph and used to estimate causal effects.
Resorting to causal graphs does not prevent the use of methods from the potential outcome framework. In this proposal, the first phase of the analysis will implement causal discovery features of graphs to recover the causal structure of the problem (i.e. the causal relations between variables). The obtained graph will then be used to select an appropriate identification strategy to estimate the causal effect, such as matching, instrumental variables or others. Following these steps, the researcher can benefit from the well-known potential outcomes literature and take advantage of a way to deal with uncertainty.
This project also proposes a novel methodology that builds on the causal graph approach. The method gives an answer to a common criticism of causal graphs: if some relations encoded in the estimated graph are wrong, the estimated causal effects could be incorrect. The new procedure uses bootstrap to identify sets of covariates for adjustment which are stable across bootstrap resamples and thus builds a confidence measure on the adjustment sets. In this way, causal effect estimation does not rely on a single graph but on a confidence measure which accounts for uncertainty in the causal structure.