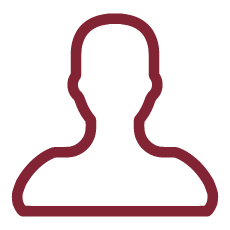
Phase-change materials (PCMs) are an important family of alloys employed in optical data storage media and non-volatile memory devices. These devices exploit the ability of PCMs to switch rapidly and reversibly between a glassy phase (logical "0") and a crystalline phase (logical "1") at moderately high temperature. At ambient conditions, both phases are very stable. These properties stem from the very strong T-dependence of the crystallization kinetics, which was attributed to the high fragility of the supercooled liquid phase and, for some PCMs, to the occurrence of a fragile-to-strong liquid-liquid phase transition (LLPT) driven by the onset of Peierls-like distortions.
Theoretical understanding of the fragility and kinetics of the supercooled liquid phase, as well as of the nature of the glass transition, requires the investigation of the structure of the potential energy landscape (PES) of the system. In this project, we will undertake this investigation for a prototypical PCM, namely the binary compound GeTe. The T=0 PES will be explored using ab-initio evolutionary crystal structure prediction, to obtain a thorough sampling of local and global minima. Then, a neural-network (NN) potential for GeTe fitted to density functional theory (DFT) results will be used to surmount the limitations in size and simulation time of ab initio methods without sacrificing accuracy. The T-dependence of the probability distribution of the local minima of the PES - the so called inherent structure energies - and their average value will be determined. This will allow us to evaluate the T-dependence of the relevant thermodynamic (configurational entropy) and kinetic (viscosity) properties, which will also yield information on the possible occurrence of a LLPT in GeTe. We will also perform a thorough analysis of the structural properties of the inherent structures to elucidate how they change with T and, thus, determine the evolution of Peierls-like distortions in the system.
This project is timely, innovative and important, both from the point of view of applications and fundamental physics. So far, MD has been used to explore systematically the PES of model systems only. In this project, we will investigate the structure of the PES of a real material exhibiting complex bonding mechanisms. This has become feasible thanks to recent advances in the construction of neural-network potentials, which provide accuracy comparable to that of DFT at a fraction of the computational cost. By carrying out this investigation for GeTe, we will shed light on a crucial property of PCMs, namely the change in the activation energy for viscosity, which yields strong temperature dependence of the atomic mobility and the crystallization kinetics. Elucidation of this property will provide guidance on a) how to further increase the crystallization speed at high temperature for ultrafast memories and b) how to increase the range of temperatures at which the amorphous state is stable for automotive applications.