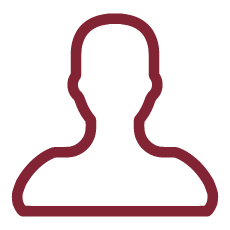
The functional connectivity between cortex and muscle during motor tasks can change after stroke, motor rehabilitation has to restore it, either by re-establishing this connectivity or supporting the development of alternative brain-muscle connectivity. Recently, as an add-on to traditional therapies, motor rehabilitation led by Brain-Computer Interfaces (BCIs) is used to improve functionality in stroke patients.
In this context, we propose cortico-muscular coherence (CMC) between Electroencephalographic (EEG) and Electromyographic (EMG) signals as a potential hybrid feature to discriminate movement tasks. The ultimate goal is to drive the development of a novel, connectivity-based, hybrid BCI system for motor-rehabilitation after stroke.
To achieve this goal, it is necessary first to identify the physiological characteristics of CMC patterns when performing simple upper-limb movements in healthy subjects. Hence, in the present proposal EEG and EMG data will be collected from 20 healthy subjects during the execution of fingers extension and grasping. The recorded data will be analysed first separately and then together, advanced approaches will be used to estimate their coherence. The EEG-EMG connectivity patterns will be studied and compared in different conditions with aim of identifying their distinctive traits. Thanks to this analysis, relevant CMC features will be extracted and used for the classification of upper limb movements.
The success in the characterization of CMC features during simple motor tasks in healthy subjects would have a wide impact on:
1) The identification of the physiological characteristics of brain-muscles functional connectivity during simple movement tasks that can be used as reference in the rehabilitation of pathological conditions such as stroke. Indeed, CMC characteristics in stroke patients might be used as objective biomarkers of stroke recovery in order to select the most appropriate/promising neurotechnology-aided intervention in each stage of the rehabilitation path.
2) The use of a complex pattern of cortico-muscular activation to discriminate different movements, building a classifier based on a hybrid feature that takes into account not only both the cerebral and muscular activity involved in the movement, but their interconnection. Hence, there is no fusion of features at the classification stage, rather it is done in the feature extraction stage. The classifier's performances will be evaluated to test its reliability, also compared with the existing classification methods. This could be relevant, not only for BCIs supporting motor rehabilitation, but also for BCI applications for motor substitution in which "natural control" (i.e. that resembling physiological control) of prosthetic devices is cutting-edge.
3) The addressing of the design of a hybrid BCI for post-stroke rehabilitation that controls devices that assist movement (e.g. Functional Electrical Stimulation, FES). Its decision to send the feedback will be based on a global vision of the physiological patterns involved in the movement that has to be recovered. This BCI-based protocol will allow to exploit the patient's residual or recovered motor abilities, giving him a feedback tailored on his rehabilitative stage. In this way, the recovery of upper limb function after stroke will be maximize thanks to this innovative hybrid BCI approach with potentially high impact on the stroke survivors' quality of life.