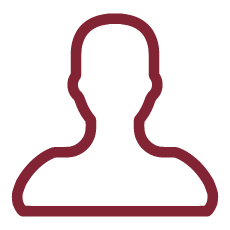
Quantum State Discrimination of multiple states is a fundamental issue in Quantum Information and Communication. The experimental realization of optimal strategies for this task is often hindered by the necessity of complex quantum receivers or supplemental resources. In this project I am proposing and implementing a scheme for multiple-state discrimination which relies on a neural network-inspired quantum receiver featuring a dynamical information processing. The experimental realization of this strategy, through an optical implementation of the quantum receiver, represents a completely new approach in view of discriminating actual quantum states. Indeed, this protocol exploits as a supplemental degree of freedom for discrimination the detection time of photons, relieving from the need for physical auxiliary resources.
Because of the network-like structure of the receiver, the implementation of Machine Learning (ML) methods becomes feasible in our framework. Through the development of adaptive protocols relying on ML, we shall be able to achieve optimal results in a wider range of scenarios through our experimental platform.
The realization of such a project will represent a completely novel approach to the problem of multi-state Quantum State Discrimination (QSD). Indeed, our scheme will be the first one to perform a four-state optimal QSD without the exploitation of auxiliary resources, since we employ the extraction time of the photons from the network as an auxiliary degree of freedom.
As such, the protocol does not require any adaptive mechanism needing a lot of signal. Indeed, this means that our protocol is suitable for applications in Quantum Communication tasks which feature actual single photon states, hence actual quantum states. Moreover, it will be the first experimental realization of a working quantum receiver featuring a network-like structure and a dynamical processing of information based on Quantum Walks, paving the way for a new vein of photonic realizations of QSD protocols.
Further benchmarking results will follow through the implementation of Machine Learning methods in our framework: we expect to achieve more general results and to experimentally realize a quantum receiver with actual neural network features.
In conclusion, our work may represent an important step in experimental and theoretical QSD strategies, proposing an alternative approach to the ones currently established, but also a starting point for even more powerful strategies, which could derive by the realization of more complex networks in the same scenario.