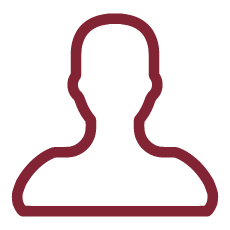
Astrophysical data sets are increasing in volume and in information density. The upward trend will continue thanks to ground facilities and space observatories, such as the ESA Ariel and Euclid space missions beginning operations within the current decade. This provides us opportunities to investigate from Galactic scales up to cosmological scales with unprecedented accuracy. Spectroscopic surveys of hundreds of exoplanetary atmospheres with Ariel will allow us to explore our Galactic neighbourhood extending, for the first time, comparative planetology beyond the Solar System boundary. The increasing accuracy of cosmological parameters estimates, a trend expected to continue with Euclid, is manifesting tensions between theory and observations. A thrilling opportunity that can be the signal of new physics, or the consequence of systematics that are no longer negligible as error bars shrink.
Astronomical data increasingly exhibit challenges shared with big data: large volumes, high dimensionality, models that are non linear in their parameters or that depend on parameters that cannot be directly constrained from observations. Making progress requires developing new data analysis techniques capable of coping with the rising data complexity.
This project aims at bringing together expertise in exoplanetary characterisation, and cosmology with galaxy clusters, lensing and CMB probes, to address the shared challenge and opportunity of large astrophysical dataset. The investigation will use observations and simulations to assess the performance of machine learning (ML) and Artificial Intelligence (AI) techniques, as well as advanced statistical methods for: i) the chemical characterisation of the atmospheres of 100s of exoplanets; ii) the estimation of the masses of cluster of galaxies needed for cosmological probes; iii) the estimation of systematics and higher order statistic properties of cosmic shear from lensing observations with Euclid.
1-Exoplanets
Our group has started a thorough investigation of the Ariel T1 dataset information content. Mock data are generated using Alfnoor (Changeat, ApJ 2020), a simulation framework developed by us in collaboration with UCL. Alfnoor combines atmospheric forward modelling with Ariel performance estimates (Mugnai, ExA 2020). A first assessment has shown that spectrally binned T1 data is effective in revealing the presence of clouds, and molecules. However, it was found that the instrumental noise biases the classification estimator, requiring a bias calibration that is difficult to obtain. Yip et al. (arXiv, 2020) independently find similar challenges applying AI techniques on higher SNR simulated Ariel data.
ML and AI techniques can be applied to T1 data to classify planets bearing certain molecules. ML+AI techniques will not be immune from biases, but can be taught how to de-bias their estimates, provided that a sufficiently large and representative example set is provided in training. We achieve this by using Alfnoor, which provides us the capability of simulating 100s of thousands mock observations and reliable noise estimates.
Therefore in this project we will
1. compare different DL techniques. KNN, MLP, RFC, SVC, CNN, etc. will be investigated;
2. assess how effective is the T1 survey is in identifying clouds and molecules;
3. extend the analysis from giants to Earth-size planets to assess how T1 data can constrain the fraction of small planets that has retained a primordial H2/He atmosphere.
The ability to identify molecules such as H2O, CO2 and CH4, chemical trends in the population and retention of a H2/He by small planets is of primary importance as tracers of planet formation and evolution (Turrini, ExA 2018; Turrini, ExA 2021).
2-Clusters
The fundamental contribution of applying AI techniques (CNNs) in clusters science is the possibility to infer total mass of a cluster without assuming previously any dynamical state. Consequently, this mass estimation is supposedly unbiased. Recent studies have shown that it is possible to infer galaxy cluster masses directly from mock X-ray images (Ntampaka et al. 2019); mock SZ images (Gupta & Reichardt 2020); CMB cluster lensing (Gupta & Reichardt 2020) and a combination of X-ray, SZ and optical mock images (Yan et al. 2020). In this project, we extend these analyses to apply for the first time deep learning in real cluster observations, such as SZ maps of Planck or X-ray maps of XMM satellites. In order to do that, we generate mock images from The Three Hundred catalogue, then we train our CNN using these simulated images and use it to predict the mass of real data. We can also compare the estimated mass inferred using our CNN with the corresponding masses estimated by weak lensing, at least for the few clusters where it is available. Moreover, clusters total mass, inferred by CNN, could have an impact on the halo mass function that is sensitive to the underlying cosmological model.
We stress that the novelty of this research is the application of ML techniques with a multi-probe approach: multi-wavelength observations of clusters of galaxies supported by state-of-the-art hydrodynamic simulations.
3-Euclid
Calibration of colour gradient bias is of fundamental importance to fully exploit the potential of cosmic shear measurements of Euclid. We will use HST data reprocessed by the Bonn Group to analyze the colour gradient properties as far as concern the bias in galaxy ellipticity induced by lensing.
The high order statistic is a new approach in the cosmic shear and convergence analysis. We investigated Minkowski functionals and Betti numbers in the case of the convergence field. Being this field not a direct observable, it is necessary to solve an inversion problem starting from the shear data. To circumvent this reconstruction problem, higher order statistics could be applied directly on ellipticity maps but the issue in this case is the lack of theoretical predictions.
For these reasons we decided to apply high order statistics on simulated noisy ellipticity maps, and to use different ML techniques to study the relation between those estimators and the cosmological parameters used to generate the simulations. ML allows us to bypass the theory issues, and it lets us directly use noisy ellipticity maps, on which we compute new higher order estimators.
The inclusion of the SSC in the computation of the Euclid covariance is a work in progress, especially for the combination of different probes coupled with the use of MCMC techniques. Computing the magnitude of the effect will allow us to quantify the degradation of the precision on the parameters' measurement and test possible mitigation strategies. The ML approach to parameter estimation from summary statistics has the potential to offer a completely different paradigm for parameter estimation and a much faster alternative to MCMC.