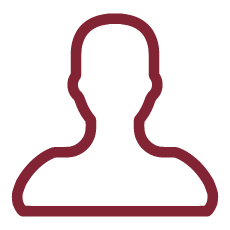
Noise prediction in turbomachinery is one of the most challenging tasks related to industrial computational fluid dynamics. In particular, accurate solutions are obtained when the boundary layer is properly resolved in the time domain, requiring fine computational grids and high computational time/resources. In addition, the derivation of the sound propagation requires the usage of specific aeroacustic solvers and grids, adding additional complexity to the process.
With this research, we propose a novel approach to turbomachinery design. Through the application of machine learning unsupervised techniques, in fact, it is possible to have an automate estimation of the sources of noise distribution in axial fans. A preliminary analysis of the problem shows that it is possible to automatically detect the sound sources on the blade surfaces by using velocity and pressure instantaneous fields. This can be achieved by means of clustering algorithms (batch kMeans). Processing the cluster analysis through big-data techniques (Dynamic Mode Decomposition) eventually lead to the dynamics of the clustering both in the frequency and in the temporal domain. Such preliminary analysis was conducted at different radial positions of the blade and based on a high-fidelity LES simulation.
This methodology can be easily extended by generating a training space of high-fidelity simulations of 2D profiles. The simulations will be conducted at different angles of attack, solidity, and using different airfoil chosen among the NACA family, commonly exploited for low-speed axial fans. The sources of noise will be computed using the Lighthill's model. The incremental kMeans algorithm will be fitted on the training dataset, with the aim of clustering different portion of the blade that show similar aeroacustic behavior. As a last step, we will apply such approach to the preliminary design of a fan to derive an a-priori distribution of the source of noises.
The proposed research shows high innovative potential. The key aspects of the research may be summarized as follows:
- Innovative design: the algorithm can be made available to the public. It may be used to have an estimation of the sources a-priori, during the design phase of the fan without additional computational costs.
- Major breakthroughs on clustering techniques for CFD: so far very few attempts are reported in literature regarding the application clustering techniques in fluid dynamics. Nevertheless, several studies show the capability of clustering algorithm to identify losses and boundary layer behavior starting from the raw flow field. Several concept may have a strong impact on future research, such as the preprocessing methodology and the selection and optimization of the different cluster algorithms.
- Alternative approach to source of noise analysis: this method can correlate different flow fields to the sources of noise. In particular, it is capable of discovering hidden correlation among variables, allowing a multi-variate non-stationary analysis of the sources.