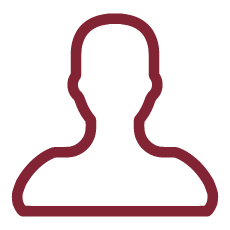
Until recently, the investigation of disease etiology, diagnosis and treatment, has been based on a conventional reductionist approach. This tenet argues that critical biological factors work in a simple linear mechanism to control disease pathobiology. Rather, they are nearly always the result of multiple pathobiological pathways that interact through an interconnected network: a disease is rarely a direct consequence of an abnormality in a single gene or molecular component. A large body of evidence that is now emerging from new genomic technologies, points out directly to the cause of disease as "perturbations" within the "interactome", i.e. the comprehensive network map of molecular components and their interactions. The unifying framework proposed by this project perfectly fits the need for integrated network-based algorithms and machine learning methods in order to reconcile biological network representation and large-scale data integration.
The project will address the following related classes of network medicine problems for precision oncology:
- Cancer genes prediction through (1a) module-based and (1b) propagation/diffusion algorithms on graphs.
- Network based methods for genome-based drug repositioning
- Network based methods for switch genes finding
The new general framework for network medicine which integrates methods from graph theory/ combinatorial optimization and machine learning, is considerably more complex and general than those used so far. The coupling of both methodologies (graph and machine learning theory and applications) is particularly suitable in the contest of network medicine for precision oncology given the large amount and heterogeneity of molecular data and the effective formulation of medical problems based on large-scale data in terms of the topology of the network, thus bringing a significant advancement with respect to results in the literature.
The new general framework for network medicine which integrates methods from graph theory/ combinatorial optimization and machine learning, is considerably more complex and general than those used so far. This complexity allows us to cover, in a mathematically sound way, different problems arising in precision oncology. The coupling of both methodologies (graph and machine learning theory and applications) is particularly suitable in the contest of network medicine for precision oncology given the large amount and heterogeneity of molecular data and the effective formulation of medical problems based on large-scale data in terms of the topology of the network, thus bringing a significant advancement with respect to results in the literature. Moreover, network representations coupled with the pattern identification power of machine learning techniques are the ideal tools for representing relationships among data, finding "patterns" for biomarker studies and, most importantly, any kind of expertise can find the opportunity to fully express itself using a common language. In fact, this working framework provides plenty of words and concepts that can be exploited by biologist/clinicians to represent their knowledge and, by bioinformaticians or computational modelers, to derive algorithms and find biological/medical answers using computation.