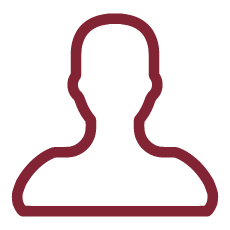
Bluetooth Low Energy (BLE) is rapidly becoming the de-facto standard for short-range wireless communications among resource-constrained wireless devices.
Initially conceived for cable replacement, Bluetooth has mostly been used for point-to-point wireless communication in the 2.4 GHz ISM band, sharing the spectrum with other technologies for local area networks including WiFi but thanks to IoT, it has found its place in low energy communications.
Nowadays, BLE is present in every mobile phone on the market and is used in almost every major field of IoT that requires efficient communication at a low energy cost, including localization, health care, audio, proximity sensing, and Covid contact tracing.
Moreover, in 2019, the Bluetooth Special Interest Group, the maintainer of the standard, formally decided to standardize the support of mesh networking, which enables many-to-many communication and is optimized for creating large-scale device networks that are suited for building automation, sensor networks, asset tracking, and any solution that requires hundreds of devices to reliably and securely communicate with one another.
Despite these promising capabilities, there is no way to date for researchers, industry, or companies to be able to study the behavior of BLE networks in the long term without actually implementing such a network with real devices.
In this project, we propose the creation of a module for the end-to-end simulation of the BLE stack to be connected with ns-3, a famous network simulator with industrial-grade specifications. This module will be based on the specifications defined by the standard and will be supported by experiments on real devices to define channel models unique to BLE and to statistically study the impact of its operations on their batteries.
Finally, this module will be made open source and will be proposed for inclusion in the ns-3 standard library to allow anyone to create their own experiments and simulations.
There are several main reasons why development in the direction indicated in this project is necessary.
In a first stance, the absence of an open-source solution to simulate these protocols will be fulfilled, enabling the research community and the industry to build on top of the proposed simulator.
Moreover, while there are a lot of collections of datasets for evaluating BLE broadcasting/beaconing mode like for instance the data collected in [1], due especially to the localization problem, there is a huge gap in the literature regarding the datasets to evaluate BLE mesh networks.
As stated before, the work in [7] of the previous section at the time of writing represents the first and only known dataset for mesh networks and this puts an enormous limit for BLE development especially in the IoT context, where to improve networks one of the most usual approaches is the data-driven.
Thanks to this proposal a lot of known and new scenarios are going to be supported, enabling the collection of the packets and the creation of huge datasets with a really high degree of accuracy.
Also, one of the hot topics these days is the security assessment of the networks.
In this field, lot of proposals are based on data analysis and while the dataset with the normal behavior of a network can be good to model a legitimate use, there is the impellent need to have datasets describing malicious action such as the drop of packets with denial of server attacks [2] or the interruption of the function of a critical service like for instance the disruption of the Friendship concept for mesh networks [3].
Thanks to the simulator proposed the generated dataset can help the development of machine learning solutions for security like for instance Intrusion Detection Systems and for optimizing the use of the battery of the device.
An example of a project that will totally benefit from this simulator is [2], in which an Intrusion Detection System for Bluetooth Mesh Networks has been developed based on pattern classification and recognition of DoS attacks such as Gray Hole attack and Blackhole attack against a server on the network. This IDS bases its misuse analysis on machine learning aiming to understand if within the network there are any attackers by creating a model able to perform a multi-class pattern classification of different traffic flows happening in a legitimate situation and when attacks are ongoing.
The data created with the simulations can be used to feed this kind of IDSs, enhancing their capabilities of detecting online attacks increasing the support for better classification.
In addition to the solutions for the presented issues, this module will open the way to many new possibilities including the possibility of large-scale simulations with more than a hundred nodes deployable at once.
Finally, the major advancement and most important will be the possibility for everyone to do an easier deployment of complex scenarios tight for Bluetooth Low Energy over established tools.
[1] Dimitrios Sikeridis, Ioannis Papapanagiotou, Michael Devetsikiotis, CRAWDAD dataset unm/blebeacon (v. 2019¿03¿12), downloaded from https://crawdad.org/unm/blebeacon/20190312, Mar 2019.
[2] Intrusion Detection System for Bluetooth Mesh Networks: Data Gathering and Experimental Evaluations 2021 - Lacava, A.; Giacomini, E.; D'Alterio, F.; Cuomo, F.
In: 2021 IEEE International Conference on Pervasive Computing and Communications Workshops and other Affiliated Events, PerCom Workshops 2021, Institute of Electrical and Electronics Engineers Inc., 2021, pp. 661-666 - ISBN: 978-1-6654-0424-2 - doi: 10.1109/PerComWorkshops51409.2021.9430966
[3] Álvarez, Flor, et al. "Toxic friends in your network: Breaking the bluetooth mesh friendship concept." Proceedings of the 5th ACM Workshop on Security Standardisation Research Workshop. 2019.