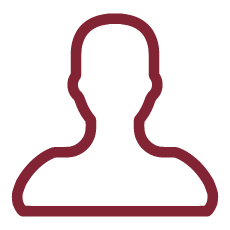
Conditional Trees (Hothorn et al., 2016) are a special case of recursive binary partitioning models where statistical tests are used in order to achieve unbiased variable selection and to solve the overfitting problem, without loss of prediction accuracy with respect to other tree-like models. So far, Conditional Trees have been used only when the nature of the variables is either nominal or numeric. We propose an extension to the case of mixed-type data, where covariates may include functional data, graphs, and persistence diagrams. The testing procedures that in Conditional Trees characterize both variabile selection and stopping criterion are here performed by means of energy statistics. Energy statistics (Szekely and Rizzo, 2013) allow to compare variables that need not to be defined on the same space, thus permitting to simultaneously model mixed-type covariates. This means that the resulting Energy Trees are a general model which can be applied to a number of cases where other models are not viable, with the additional advantage of being strongly based on statistical testing procedures. Preliminary results obtained in both simulated scenarios and real-case analyses are promising, and definitely foster further explorations in the area.
References:
- T. Hothorn, K. Hornik and A. Zeileis (2006). "Unbiased Recursive Partitioning: A Conditional Inference Framework", Journal of Computational and Graphical Statistics, 15(3), 651-674.
- G.L. Szekely and M.L. Rizzo (2013). "Energy Statistics: A Class of Statistics Based on Distances", Journal of Statistical Planning and Inference, 143(8), 1249-1272.
This project's main goal is to develop a systematic framework for a model which is general enough to receive as input and accommodate almost any kind of covariates. Up to now, no working attempt to do so is known by who is writing. The innovation in the field of supervised learning would be of huge impact. Obtaining such a general model means that almost any analysis in this research area would be possible, without the need of transforming complex variables in order to have data in the traditional (nominal or numeric) format.
The potentiality to reach this goal is concrete since some minimum working examples have already been obtained separately both for functional data and for graphs, leading to satisfying results in comparison with other models. Next step is to simultaneously include in the model these two types of data, and then to extend the procedure also to data of different kinds.