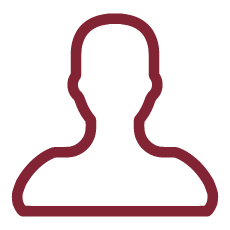
In composite indicators construction the robustness analysis is an important step suitable to validate the synthetic measure and to attempt to evaluate the loss of information.
This paper presents an interval between the best and the worst performance obtainable from the synthesis of a multi-indicator system, useful for the robustness analysis of composite indicators. The upper bound of this interval is obtained by using the Benefit Of The Doubt (BoD) approach and the lower bound is equal to the minimum between the basic indicators considered. The obtained range includes almost all synthetic measures that can be calculated with aggregative methods and it is suitable to analyze the distance of different values obtained by means of different methods from the best and the worst obtainable ones.
The mean value between the extremes of the interval is proposed as a new aggregation method. This medium point (MP) presents some interesting properties. It can be considered as only partially affected by compensability; in fact, it is exactly in the middle between the extreme calculated with the BoD, that is partially affected by compensation, and the minimum, that is totally non-compensative. Moreover, this point is the center of a symmetrical interval and, consequently, it gives an easily observable representation of the distance from the best and worst value obtainable from synthesis.
At the end, we propose a method to verify how properly a chosen synthetic method represents the structure of the data used by using model based clustering via finite Gaussian mixture models.
Assuming that the model based cluster analysis performed gives the best possible representation of the distribution of the data and assessing that every synthetic measure could be seen as an ordinal variable, we can evaluate the ordinal synthetic measure according to the miss-classification that it gives of the discriminatory capacity of the model based clustering.
The novelties of the research are: The BoD - min range of performance and the synthetic measures validation method via finite mixture model and model based clustering, as new tools for the validation and the choice between synthesis methods of multidimensional data. And the medium point between the extremes of the BoD - min range as weakly compensative aggregation method.
The BoD - min range allows to make the range of variation of each unit narrower or, in extreme cases, equal to that of the normalization method Min-Max, using the BoD method to calculate the upper bound of each unit and the minimum between the basic indicators for limit each unit below.
The first advantage of this method is the calculation of a range of variation of the performance for units, given by the observed values and in which each point is hypothetically reachable.
Given a set of indicators normalized with the Min-Max method, we define a new interval, in which every point is a possible result of the synthetic indicator of the considered unit, for all the most used aggregative methods.
From the first point, it follows the second advantage of our interval, that is to have, in the synthetic indicator, a real and specific range for each unit. Within that range which, the results of most of the most commonly used aggregation methods are included. Consequently, this makes them comparable with respect to the maximum and the minimum level achievable by the unit for each basic indicators considered.
The third goal of the proposed method is that allows the evaluation of each single unit not only relatively to the others, but also to itself, by comparing the level reached with the chosen aggregation method with the calculated upper and lower bound.
Whichever aggregation method is chosen in the interval, we can divide the space within the proposed range into several parts and easily calculate the sets of weights needed to reach the upper subspace. The logic behind this, is to give to each unit different sets of possibilities (weights) that allow them to make decisions to gradually approach the hypothetical maximum achievable represented by the upper bound (BoD).
The medium point between the two bounds, it can be chosen to represent the status of the units in the synthetic measure.
The medium point can be considered as only partially affected by compensability; in fact, it is the middle point between the extreme calculated with the BoD, that is partially affected by that, and the minimum, that is totally non-compensative. The main quality of this aggregation is that the point found is at the center of a symmetrical interval and, consequently, it gives an easily observable representation of the distance from the best and worst case.
At last, the proposal of synthetic measures validation method via finite mixture model and model based clustering is an attempt to answer the question: if each method has pros and cons, how is it possible to asses that the used method gives a good representation of the data matrix?
The answer given is that a good synthetic measure, should give a good fit of the distributive assumptions made on the data. That means that a good aggregative method should lead to a synthetic measure that gives a good representation of the distributive form, or the multiple forms, that we have assumed on the data matrix, and therefore a good aggregative method should transfer as much as possible of the original information in the synthetic measure, without altering it. The next problem is how to understand if a synthetic measure gives a good representation of the distributive assumptions. After having assumed one or more distributions on data, we can use it to classify the units into groups, through a model based clustering, therefore for each unit we will have the cluster to which it belongs. Assuming that the model based cluster analysis performed gives the best possible representation of the distribution of the data; if the synthetic measure used respect properly the clustering discriminatory capacity, we can asses that the chosen aggregative method is a good choice.