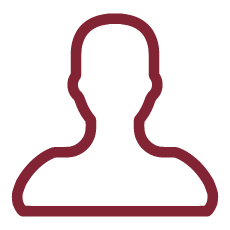
The incidence of differentiated thyroid cancer (DTC) is rising. It has an excellent prognosis, with low rates of recurrence and mortality. The clinical challenge is to personalize treatment, reducing the burden of unnecessary testing and treatments. The prerequisite is a correct classification of patients according to their true risk of recurrence.
A three-tiered risk system was proposed by the American Thyroid Association to predict the risk of structural disease recurrence. In this system, the calculated risk is based on selected clinico-pathological features.
Some molecular data may be used to refine the risk-estimate: BRAF V600E and TERT mutations are included
in the revised version of the system. Even if BRAF V600E mutations are frequently reported in a subgroup of PTCs with more aggressive clinicopathological behaviours, the need for routine genotyping of PTCs has not been established.
To clarify the contribution of molecular signatures to the risk stratification of DTC, we plan to employ the web-based thyroid cancer database of the Italian Thyroid Cancer Observatory (ITCO) (prospective, contemporary observational data) and novel technologies such as machine learning and artificial intelligence.
Using a prospective dataset, including baseline data and actual outcome of patients three and five years after the initial treatment, we can estimate the accuracy of the risk stratification in a population with DTC and how it may be improved and modified by the availability of a comprehensive molecular profiling. Employment of innovative technologies will also allow for identification of unknown risk predictors.
The final clinical aim is allowing an accurate risk assessment to be used to guide initial prognostication, disease management. A proper risk assessment will minimize overtreatment in the majority of at low-risk patients and will appropriately allow for treating and monitoring those patients at higher risk, in a cost-effective way.
Large multicenter and prospective studies on proper evaluation of risk assessment in thyroid cancer patients are missing. The web-based thyroid cancer database of the Italian Thyroid Cancer Observatory (ITCO) is an interesting and growing dataset that may be explored using novel technologies such as machine learning and artificial intelligence. ITCO database includes prospectively updated, observational data on a consecutive series of more than 8000 patients with newly diagnosed thyroid cancer. The median of the follow-up is 15 months (range: 3-89 months) with an enrolment rate of approximately 130 new patients each month.
The collation of clinical, pathological, molecular, along with the final outcomes detected during long-term follow-up will allow a revision of current assessment strategies for prognostication, disease management, and definition of follow-up strategies.
A contemporary, up-to-date, prospective, and multicenter dataset of thyroid cancer patients is essential to apply a machine learning approach to accurate real-world data, letting the artificial intelligence to learn from current clinical practices. The proper risk assessment is of paramount importance to minimize overtreatment of low-risk DTC patients, focusing treatment and monitoring efforts on those few patients at higher risk of recurrence or cancer-specific mortality.
The employment of machine learning will allow for identification of unknown risk predictors and to estimate the real clinical usefulness of genomic and molecular data. Finally, it will clarify if the expensive genomic analyses are effective in real clinical practice and provide clinically-relevant prognostic and predictive markers. Furthermore, it will integrate all available information in a comprehensive scoring system.