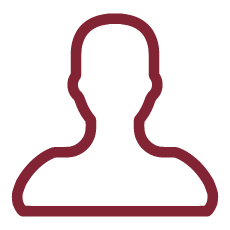
Multiple sclerosis (MS) is an inflammatory and neurodegenerative disease that represents the main neurological cause of disability in young adults. Clinical outcome in all forms of MS is highly heterogeneous. Due to the uncertainty of the disease course, decision about the best therapeutic strategy in the single patient may be difficult. The identification of disability predictors requires complex mathematical models able to combine large amounts of longitudinal data.
The aim of this project is to use deep learning approaches in order to: 1. classify patients with 5-year disability progression on the basis of T1 and T2 MR images and 2. evaluate which combination of clinical features and MRI changes has the highest prediction accuracy.
The study will include 250 MS patients who already underwent clinical and MRI 3T evaluation between 2010 and 2015 and a 5-year clinical and MRI follow-up (between 2015 and 2020). Disability progression will be assessed according to Rio et al. (2006). Various MRI measures of brain structural damage, as lesion load, global brain volume, cortical thickness, thalamic, cerebellar and cervical cord volumes, mean fractional anisotropy of the apparently normal white matter, will be obtained. For each MRI measure, we will estimate changes at the follow-up with respect to baseline.
Two different machine learning approaches will be used: 1. to classify patients with disability progression in the following 5 years (aim 1), we will investigate T1 and T2 MR images by optimizing Inception-V4 pre-trained models and Tensorflow library (Google Inc). 2. To determine which clinical/imaging features weight more on disability progression (aim 2), we will combine in a neural network imaging features, i.e. changes in above-mentioned MR parameters, and clinical features (age, gender, phenotype, disease duration, EDSS at baseline, number of new relapses during follow-up).
Although deep learning algorithms have been used to build classifiers in the field of MS research, and were able to accurately classify MS patients and healthy controls, as well as different MS phenotypes, no study has applied so far MRI-based deep learning algorithms to classify disability on the basis of a long-term follow-up.
Given the high heterogeneity of disease progression in all forms of MS, the therapeutic choice requires a personalized medicine approach.
By using MRI-based deep learning algorithms, this project aims at providing clinicians with a tool to identify individual cases at risk of disability progression.
By evaluating MRI changes after a follow-up period and their impact on disease progression, this study may provide insight to pathophysiological mechanisms of MS. Overall, by determining MRI predictors for disability progression in MS via deep learning algorithms, the results of this research may help to decide the best therapeutic approach in individual patients. Medical decisions, practices, interventions will be tailored to the individual patients based on their risk of progression.
Preliminary results of our lab on a series of 105 patients with MS indicate that this project has high probability to be successful.