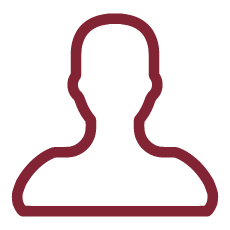
[1998 chars (max 2000)]
Taking the right decision in safety-critical clinical domains is a complex task of the utmost importance.
For example, choosing psychotropic drugs for mentally ill pregnant/lactating women (our first application) involves the wise weighting of many factors. A wrong choice could lead to severe consequences, e.g., embryo malformations, suicide, infanticide.
Similarly, in the forensic field (our second application), psychiatric evaluations of the criminal accountability or social dangerousness of a defendant involve a multi-factor evaluation of the subject. A wrong assessment could end up with the criminal punishment (e.g., jail) of a mentally ill patient (who should deserve psychiatric care), or, vice versa, the psychiatric treatment of a defendant who should be punished.
Despite their critical nature, such decisions are taken by (usually individual) experts in a context of a general lack of standards.
This project aims at supporting the quantitative modelling and validation of both the peculiar strategies of individual experts as well as those pinpointing the aspects most agreed-upon by the entire experts¿ panel.
Such strategies will be exploited to:
1. Analyse in silico the similarities and differences of decisions of our experts, support evidence-based discussions, promote uniformity and self-awareness of personal biases;
2. Support design and prioritisation of ethically-principled prospective trials (e.g., experimental strategy vs. treatment as usual) to assess the quality of the learnt strategies, sustaining the emerging of best practices and golden standards;
3. Power Decision Support Systems (DSSs) for evidence-based decision making in those fields.
Our hybrid approach combines knowledge-based AI, knowledge engineering, machine learning, as well as active and interactive learning to generate synthetic (i.e., virtual) patients, plus gamification to leverage the presence of several domain experts in our interdisciplinary team.
[4986 chars (max 5000)]
== PI's working time allocation to the project ==
As requested by the Sapienza regulation, the PI hereby declares he will allocate 33% of his working time to this project (12 PM in 3 years).
== State of the art ==
Currently deployed DSSs in psychiatric and forensic domains suffer from lack of flexibility, usability, and knowledge sharing capabilities [30]. Furthermore, the knowledge acquisition process [13], most often based on direct elicitation of requirements from experts, is slow and cumbersome, and the rules obtained typically do not duly consider all the possible exceptions.
As a result, suggestions offered by current DSSs often address typical cases only, and do not take into proper account the peculiarities of each case (e.g., patient¿s co-morbidities), which would actually have a strong impact on the best decision (e.g., the most suitable therapeutic choice).
Active [27] and interactive learning [4] are promising paradigms to overcome some of the obstacles of current approaches to DSSs design. These approaches aim to perform supervised ML by intelligently [11] synthesising their training set on-demand, and by exploiting the availability of experts for labelling training instances, thus regarding them as human oracles [16].
The basic and simplistic approach of assuming a single infallible, consistent and always available oracle has been extended to handle multiple noisy oracles [24, 19, 3]. Also, extensions to integrate prior expert knowledge acquired from retrospective data [33] or logic rules [25, 20] have been proposed.
Furthermore, to make the knowledge acquisition process more effective in practice, gamification approaches have been widely used in requirements [6] as well software engineering [23]. Lately, also the world of digital healthcare has experienced the use of serious games, but, differently for our case, most attempts focus on non-safety-critical domains, such as general training medical students and patients¿ self-monitoring [15, 26].
== 3.3 Envisioned progress beyond state of the art ==
We aim at advancing the state of the art in the directions below.
**Serious games mechanics and user engagement**
For our platform to be effectively and intensively used by our players, the user interface must be highly usable, and the envisioned game mechanics must be highly engaging. In particular, the involvement, in the validation/refutation of formalised rules, of domain experts with a cultural background very far from mathematics and computer science requires innovative methods to present them rules and constraints in forms, e.g., graphical or narrative, they can immediately understand and modify, if needed.
**Domain knowledge acquisition via active learning**
We will advance the state of the art in active and interactive learning, by providing effective methods for the continuous acquisition and validation (via our serious gaming platform) of domain knowledge by means of independent domain experts.
**Hybridising ML with knowledge-based AI**
ML from data is popular nowadays, but suffer in those situations where available training data is scarce, where explainability is a must, and where the presence of biases in the learnt models cannot be tolerated.
In safety-critical settings such as ours, such weak points of ML are amplified. Indeed, gathering massive amounts of retrospective data is extremely difficult in our target domains, and such data are often only partially representative of the whole spectrum of the situations that can occur in practice. Furthermore, lack of explainability of the reasoning that led a DSS to the provided suggestion hinders their adoption.
We will advance the state of the art by devising methods that allow a sound hybridisation of ML algorithms with knowledge-based approaches, in order to enforce suitable constraints (directly coming from domain knowledge) onto the learnt models and support explainability and biases removal.
**Applications**
In both our domains, decision making is performed in a general setting of lack of established standards. This is because randomised trials would be difficult to carry out, if not simply impossible due to ethical reasons. Hence, most available knowledge is anecdotal or comes from purely observational studies. Evidence exists that decisions in these fields, although critical, are often too much subjective and suffer from severe cultural or cognitive biases of the decision maker. Well known examples are: stigma against severely depressed pregnant/lactating women, and biases (e.g., racial, sexual) during forensic psychiatric evaluations.
Our serious gaming platform will enable, for the first time in both areas, a safe, controlled, in silico environment where to perform evidence-based analyses of the approaches currently adopted by decision makers, fostering confrontation and emerging of the best practices, as well as supporting domain experts to improve self-awareness of their own biases.