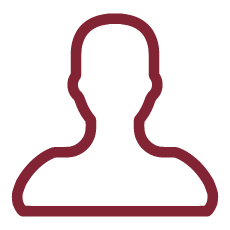
Traditionally Machine Learning (ML) assumes training data is centrally available in big data centers. This assumption does not always hold in real-world applications.
Federated Learning (FL) allows training ML models by combining decentralized training data in a privacy-aware fashion, i.e., without the need for physically exchanging the data itself. State-of-the-art federated learning approaches, though, are designed for supervised learning, thus relying on expertly labeled data, which is costly and time-consuming to obtain in large quantities. For example, for automatic detection of carcinoma cells, public datasets are composed of a few hundred images, which is multiple orders of magnitude less than other domains. Recently, self-supervised models have gathered wide popularity in the literature as a potential solution to this issue, by reducing the reliance on fully labeled data and opening up the possibility of training Deep Neural Networks (DNNs) by relying almost exclusively on unsupervised data. However, very little is known about the performance and the theoretical guarantees of self-supervision in FL scenarios. To overcome these issues, the aims of FedSSL are twofold. First, we plan to design a fully self-supervised, FL algorithm for DNNs, allowing it to be efficiently trained from large quantities of unsupervised data distributed across multiple actors. Second, the algorithm will be thoroughly analyzed from a theoretical standpoint to understand its convergence guarantees, communication requirements, and generalization performance.
The model will be tested on a realistic medical use case, by training on a newly collected large unlabeled dataset of urinary bladder cancer, one of the leading death causes for men in Italy. Data and models will be publicly released to additionally foster research in these domains. The application to the medical domain will also allow testing the feasibility and the performance of self-supervised approaches to the medical domains.
Over the last years, deep learning solutions applied to medical imaging have received widespread interest, with a significant number of works published from diabetic retinopathy to cancer detection, handwriting recognition, and functional MRI analysis. However, only a very small percentage of these works have gone successfully from a prototype in a research laboratory to a production environment [ROB21], thus limiting the practical impact of the research. As mentioned above, the two major obstacles to this end have been the limited amount of labeled data on which these models were trained and, in several other cases, a mismatch between the domain on which the models were trained (collected over a short period of time from a single institution) and the scenario on which the models were asked to make a prediction.
FedSSL aims at overcoming both shortcomings, thus increasing the amount of deployed deep learning models in medical domains and reducing the gap between research and development. Apart from the urinary cytopathology use case we plan to explore, the algorithms will find application in a wide range of critical tasks where data is currently shared among multiple institutions and is only partially annotated (e.g., volumetric data for the analysis of fMRI scans).
Having a federated learning model will allow multiple institutions, geographically separated, to share their data with no concern about the privacy of the patients, while having access to self-supervised algorithms is the key to exploiting all the data (even unlabeled one) present in the medical databases. Access to a well-curated repository with the developed algorithms and use-case is also expected to foster additional research and accessibility from other researchers.
More in general, the development of federated algorithms for self-supervised models will have a wide impact on several other fields of research. In particular, federated learning was originally designed to access personal data on smartphones for training models by never directly sharing the data itself. However, only a very small percentage of data collected by a user on a personal device is labeled (e.g., photos, videos, speech, motion sensors). Recent research on self-supervised models has shown that exploiting this unlabeled data for pre-training models can provide significant boosts in accuracies, especially in low-resource domains (e.g., speech recognition in Italian) and for low-represented classes (e.g., detection of very rare objects).
The healthcare application that we are envisioning offers a particularly good use case for Self supervised FL. First, and foremost, labelled data is usually extremely scarce in this domain. Medical data are sensitive by nature and they cannot be shared even among different labs or hospitals; data is relatively rich (high resolution images) and the performance of models is important.