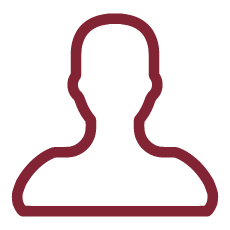
The unique historical-cultural heritage within the urban area of Rome is affected by different geo-hazards, thus determining high risk conditions. This project aims at characterizing, modeling and verifying the spatial-temporal occurrence and rate of geo-hazards which interact with the geological urban setting, in order to give contributions to preserve its value and assets by making it safer and resilient through proper and sustainable risk mitigation strategies. To account for the characteristics of some of the geo-hazards affecting the area of Rome (i.e. subsidence, flooding, landslide, sinkhole, seismic and related effects) and their interactions in the urban context, a multi-approach study rationale will be adopted involving a comprehensive urban geology and geo-hazards knowledge, monitoring and modeling of ground and underground instabilities and potential domino effects. The research will consider the implementation of tools and smart solutions for multi-geo-hazard analysis.
The project is structured in 3 Work Packages (WP):
1) database design and implementation,
2) ground instabilities, seismic and flood hazard analysis,
3) future hazard scenarios modeling.
Databases, models, tools and methods will be demonstrated with prototype implementation in specific areas of the pilot city of Rome which presents pressing challenges such as urban sprawl, ground instabilities, seismic and flood hazard. The impact of each geo-hazard as well as combined and domino effects will be examined and assessed in view of their utilization for the Resilience Strategic Plan of Rome.
Due to the growing need to remotely manage the territory and its assets, predictive models able to combine geospatial DB, which return as outputs qualitative and/or quantitative geological multi-hazard ratings, would have an impact not only in the technical field (e.g. for assets managers and insurance companies), but also in the scientific field, as it would be an important advance in understanding the interrelations between different geological hazards and their dependence on weather conditions and anthropic factors.
To reach this objective, the project is intended to take advantage of the latest spatial data science techniques in order to catch hidden patterns between data and to develop probabilistic multi-hazard maps.
One of the most innovative aspect of the project is represented by the integration and cross-validation of various geospatial data formats and sources in a single geo-database. Once the geo-database will be completed, the process of analysis and assessment of hazard levels for the city of Rome will be carried out through classification and/or regression algorithms that belong to the Artificial Intelligence domain. Artificial Intelligence is the base of the pyramid. In the present project we will exploit a subset of AI, that is Machine Learning. Machine Learning (ML) is the actual process the machine uses to learn, so the AI can make decisions. By exploiting historical data, Machine Learning algorithms represent therefore the most promising technique for predictive models concerning natural hazard assessment and risk reduction.
Unlike conventional geo-hazard maps that are pixel-based and often homogeneously distributed over the whole territory, taking advantage of the information available on the various infrastructures existing in the city of Rome, multi-hazard maps developed in this research project will have a city-structure perspective. This means that each urban structure will have a value of hazard for each threat coupled with a synthetic value regarding the multi-hazard rating. Moreover, an important step forward with regard to the actual state of the art is represented by the use of graph theory for the creation, manipulation, and study of the structure, dynamics, and functions of complex networks as the ones in the city of Rome. With this regard, urban assets will be linked to each other, thus helping to classify assets on both geological hazards and the role of each asset in the network, resulting in a network-ranked hazard zonation. The results will be displayed both as hazards zonation maps and in a Geo-hazard Assessment Grid where, given an area of interest, a summary of the probability, intensity and interference with urban structures will be reported.
Being able to accurately predict geological hazards in urban areas is a challenge many insurance companies face today. Predictive modeling can be a useful tool for early analysis and assessment of ground instabilities for vulnerable communities. Reducing risk with machine learning will have important applications concerning the improvement of sustainable development strategies and the predictive maintenance of urban assets and historical-cultural heritage, which would lead to better risk-informed decision-making to help save lives, reduce asset life-cycles costs, and protect the environment.