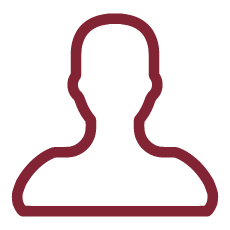
Existing group mobility models were not designed to meet the requirements for accurate simulation of current and future wireless networks scenarios, that need, in particular, accurate, up-to-date information on the position of each node in the network, combined with a simple and flexible approach to group mobility modeling. This issue is particularly relevant for 5G and beyond 5G mobile wireless networks, where small scale variations in relative distance between terminals may have a severe impact on the radio communication channel: existing group mobility models are inadequate under such conditions, since they do not
model the individual mobility patterns of each node.
The goal of the GMOMO project is address this issue by first defining a new framework for defining the properties of group mobility models in terms of relationship between network nodes; this framework will be then used to introduce a model for group mobility in wireless networks that is capable of accurately describing all mobility scenarios, from individual mobility, in which nodes move independently one from the other, to tight group mobility, where mobility patterns of different nodes are strictly correlated.
The project will also define a new set of performance indicators for group mobility models, that will allow a quantitative analysis and comparison of the proposed model with preexisting models. The comparison will consider realistic scenarios for 5G and beyond 5G networks, e.g. massive mmWave and Terahertz networks, as for example a set of mobile nodes cooperating in the realization of a distributed MIMO link.
The final result of the GMOMO project will be the definition of a new accurate, robust and flexible model for micromobility of groups of nodes in the wide range of network scenarios expected to characterize 5G and beyond 5G networks. The source code of the model, suitable for adoption in existing discrete event simulators, will be also made available under an open source license.
The achievement of each of the goals stated for GMOMO in the previous section will in turn lead to an advancement beyond the state-of-the-art in the field of group mobility modeling, as detailed in the following.
1) definition of a framework for the characterization of group mobility models - currently, mobility models are mostly defined with a specific mobility scenario in mind. The Pursue and Column mobility models, for example, target two well defined mobility patterns. The framework to be defined in GMOMO will unify the description of these and many other mobility models, by introducing a data structure capable of describing the relationship within nodes. The definition will move from the idea of a binary group relationship matrix describing the relationship between nodes, covering all models between the two extremes of a purely individual mobility pattern (identity matrix) and a pattern where all nodes belong to the same group (full matrix); the data structure will be furthermore time-varying, in order to cover scenarios where group relationships vary over time.
2) introduction of a new dynamic group mobility model - the model to be designed in GMOMO will be defined within the framework for the characterization of group mobility models, and will move from previous work carried out by the proposer for group mobility modeling originally presented in [20]. GMOMO will go beyond such previous work by introducing collision and obstacle avoidance properties similar to those currently available in behavioural models, but only based on relative speeds, directions and position of nodes, without introducing the concept of repulsive/attractive forces that makes behavioural models hard to apply. Overall, the model will combine an advanced individual mobility model with dynamic, flexible management of groups relations, going clearly beyond what is currently available in the literature.
3) define a set of key desired properties for a mobility model, and corresponding performance indicators - The most straightforward approach for evaluating a mobility model consists in comparing mobility traces collected in real world with the traces generated by the model; unfortunately, mobility traces for groups of users/devices in a micromobility context are not available in the literature. Mobility trace databases are in fact almost exclusively available for large scale mobility, e.g. large fleets of military vehicles [21], civilian vehicles [22], or human mobility over daily/weekly periods [23], [24]. The alternative approach to compare mobility models has always been to assess their impact on network protocols; although this may provide interesting insights, it is hardly an effective way to determine the actual quality of the mobility model itself. The definition of a set of intrinsic properties of a mobility model will provide an objective way of comparing mobility models.
4) extensive performance evaluation of the group mobility models - the use of the proposed set of metrics will allow to test group mobility models as a function of both model parameters and discrete event simulator settings: this will allow to determine for each model whether it can be adopted in all simulation settings, or if it leads to artifacts (e.g. impossible speeds) in specific scenarios, an aspect that is typically neglected in performance evaluation.
5) open source release of c++ code implementing the proposed model and state-of-the-art models - this release will allow reproducibility of results, and support further research on group mobility models for micromobility.
[20] Luca De Nardis, Daniele Domenicali, and Maria-Gabriella Di Benedetto. 2010. Mobility model for Body Area Networks of soccer players. In European Wireless Technology Conference (EuWiT). 65-68.
[21] Yung-Chih Chen, Elisha Rosensweig, Jim Kurose, and Don Towsley. 2010. Group Detection in Mobility Traces. In Proceedings of the 6th International Wireless Communications and Mobile Computing Conference (IWCMC ¿10). ACM,
New York, NY, USA, 875-879.
[22] Y. Li, M. Zhao, and W. Wang. 2013. Internode Mobility Correlation for Group Detection and Analysis in VANETs. IEEE Transactions on Vehicular Technology 62, 9 (Nov 2013), 4590-4601.
[23] I.O. Nunes, P.O.S. VazdeMelo, and A.A.F. Loureiro. 2016. Group mobility: Detection, tracking and characterization. In 2016 IEEE International Conference on Communications (ICC). 1-6.
[24] M. Suzuki, T. Kitahara, S. Ano, and M. Tsuru. 2018. Group Mobility Detection and User Connectivity Models for Evaluation of Mobile Network Functions. IEEE Transactions on Network and Service Management 15, 1 (March 2018), 127-141.