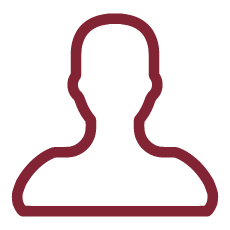
Housing is a topical and complex issue both in social and economic terms. It highly affects household well-being and it could have a great effect on increasing or reducing households standard of living. Several works analyse housing in relation to inequality and they have highlighted the difficulties to include non-monetary components, mostly housing benefits. The purpose of this research proposal is to analyse the impact of housing on poverty and inequality in several aspects. The main questions that arise about housing are the following:
1. What is the best approach on computing housing into equivalised household disposable income in order to measure income inequality?
2. How housing affects inequality and vice versa: social and economic implications
3. Which data are more suitable to analyse housing? Surveys, administrative or 'big data' database.
4. Taxation, public incentives and housing market: the role of the 'Ecobonus 110%'.
5. Which is the effect of rising/reducing house prices on inequality?
6. New technologies and challenges: which is the effect of sharing economy platforms (e.g. Airbnb, Booking.com) and SARS-CoV-2 on housing market?
The right to housing is recognised in the Universal Declaration of Human Rights of 1948 and in the International Covenant on Economic, Social and Cultural Rights (1966). In both documents housing is linked to the right to an adequate and decorous standard of living. Analysing how housing contributed in increasing or decreasing inequality, especially during shocks, is intriguing for policymakers and for academic purposes. The potential use of big data could become an interesting source of information, which can be used for detailed researches. Understanding the dynamic of housing market in several aspects could help policymakers to correct distortions and to implement policy evaluation.
Dataset used in most of the literature concerning housing and income inequality topic are survey data (e.g. Filandri & Olagnero, 2014; Dewilde & Decker, 2016; Dustmann et al., 2018), especially data from European Union Survey on Income and Living Condition (EU-SILC). Anyway, in other kind of analysis, different dataset are needed. Recent studies (Loberto et al., 2018; Baldominos et al., 2018) exploited the potential of big housing data in their analysis, using online portal for real estate market, in order to fill the gaps of administrative data on housing market, especially the absence of detailed physical characteristics for house sold. They built the dataset using housing sales advertisements and then they correct a distortion due to multiple advertisements for the same unit using machine learning tools. The information provided are several, both quantitative and qualitative: price, number of visits (clicks) and contacts on the advertisement, physical and numerical information (rooms, bathrooms, air conditioning, energy class etc.), contractual, geographical and temporal characteristics. Once the dataset has been constructed, multiple analysis can be conducted: the evolution of housing sector - even at micro regional level - and the dynamics of prices, preferences of the users provided by the number of clicks (in particular after SARS-CoV-2). These kind of analysis cannot be conducted with other existing and public datasets.
However, administrative data can also be useful in an housing market analysis. In Italy the main provider of data on housing is Osservatorio del Mercato Immobiliare (OMI) of the Italian Revenue Agency (Agenzia delle Entrate). These dataset are particularly suitable in order to conduct taxation analysis, which is a topic intrinsically related to inequality, both at income and wealth level. Another analysis that could be conducted is related to the energy efficiency of housing market and the role of public incentives: the so-called "Ecobonus 110%" in Italy (D.L. n. 34/2020 "Decreto RilanciO").
REFERENCES:
BALDOMINOS, A., I. BLANCO, A. J. MORENO, R. ITURRARTE, O. BERNÁNDEZ AND C. ALFONSO (2018): Identifying Real Estate Opportunities Using Machine Learning, Applied Sciences, 8, 2321
DEWILDE, C. AND P. DE DECKER (2016): Changing Inequalities in Housing Outcomes across Western Europe, Housing, Theory and Society, vol. 33, issue 2, 121-161.
DUSTMANN, C., B. FITZENBERGER AND M. ZIMMERMANN (2018): Housing Expenditures an Income Inequality, CReAM, University College London.
FILANDRI, M. AND M. OLAGNERO (2014): Housing Inequality and Social Class in Europe, Housing Studies, 29:7, 2014, 977-993.
LOBERTO, M., A. LUCIANI, M. PANGALLO (2018): The potential of big housing data: an application to the Italian real-estate market, Banca d'Italia WP n. 1171