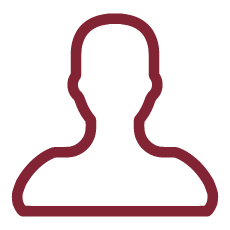
In recent years, there have been important advances in the ability of precisely reconstructing the 3D face of persons starting from simple photographs (see, as an example, Deng, Yu, et al. "Accurate 3d face reconstruction with weakly-supervised learning: From single image to image set."). However, for many surgical and legal-medical applications, the reconstructed information is not sufficiently complete and detailed. In this project, we aim at investigating the feasibility of extending the current state of the art to significantly more complete models of the human ear that include the bones and the soft tissues,.
We plan to accomplish this research task by putting together the competencies of maxillofacial surgeons and computer science specialists so to:
1 Assemble a dataset composed of images, 3d reconstructions, and CT scans including people with head traumas and pathologies.
2 Develop anatomically accurate models of the human head that includes normal and pathological patients, that can be described by anatomical and genetic parameters.
3 Using Artificial Intelligence and deep learning techniques, implement neural networks-based software tools that will allow us to reconstruct, starting from simple images, the complete structure of the head.
4 As a second step, this will be the basis of a tool for the support of the identification of people from face images.
We now detail the tasks composing the project:
Task1: The project's first task will be an extensive analysis of the models and the methodologies present in the literature for 3D face reconstruction. The main focus will be on the study of state of the art regarding deep learning approaches. This kind of approach makes it possible to lower computational, hardware, and technical skill requirements at implementation time. Despite that, this class of methods is still relatively new and has not yet reached the accuracy and versatility of photogrammetry and 3d. Furthermore, direct comparisons of deep learning methods to classical approaches are sparse. Therefore, the first step will be a thoughtful evaluation of the performance of the neural network models present in the literature compared to photogrammetry and 3d scanning.
Moreover, differently from other methods, Neural networks require a training process on datasets of relevant size. This poses several challenges as the acquisition of data and, in particular, of 3d face scans is an expensive endeavor both economically and time-wise. Another investigation point of this first task will be the study of currently available datasets. This step will also explore the possibility of exploiting medical data such as DICOM to produce data samples suitable for the deep learning pipeline.
Finally, as the project aims to produce an easy to deploy architecture, we will also explore the hardware requirements of currently available methods. The knowledge acquired in this step will provide a useful guideline regarding the software constraints that the architecture will have to respect to keep the hardware specifications low.
Task2: This task includes the planning and development of a new database that will contain all the information relevant to the project. The precise design of the database will be decided during the course of the project, but it will include all the raw and processed data relevant to our research.
The proposed database will contain information both on normal persons and on persons with head traumas and pathologies. The information will be obtained from datasets available for scientific research as well as from the partners of the project. All information will be acquired in respect of the Italian privacy laws.With the current widespread use of 3D facial surface imaging in clinical and research environments, there is a growing demand for high-quality craniofacial norms based on 3D imaging technology.
Task3: Another purpose of this project is to assess the existing best practices in the segmentation of medical images deriving from MRI (Magnetic Resonance Imaging) and TC (Computerized Tomography) in order to define a general scenario for the extraction of the craniofacial structures or head pathologies. The most robust techniques will be evaluated on test cases and a multi-method strategy will be defined to create a 3D labeling algorithm that encompasses the information coming from individual segmentation strategies.Methods based on the similarity between voxels (3D pixels), based on gradient information, and based on the level-set framework will be taken into account. With the help of the anatomists, participating in the project, average shapes and morphological variability of different anatomic structures will be defined, with a specific focus on the bone tissue, muscular structures, articular sub-structures, and the entire shape of the head. The creation of a database of prototype shapes will allow deploying advanced level-set methods based on a shape prior, where the algorithm extracts the desired tissue with a clear notion of the final shape that the segmented portion of a 3D volume should assume, given that a specific structure is sought after (e.g. temporal bone).
Then, the goal of this activity is to survey the existing literature to assess the availability of segmentation methods based on gradient information. Differently from the region-based segmentation, which produces a sub-volume of labeled voxel directly, the gradient-based strategies define a boundary surface that contains the segmented sub-volume. In this category fall the edge-detection/edge-linking methods together with the snake (deformable/active contours) approaches.
Task 4: Development of new and more general reconstruction algorithms. Test of the new models with the developed dataset and with new data.